Papers
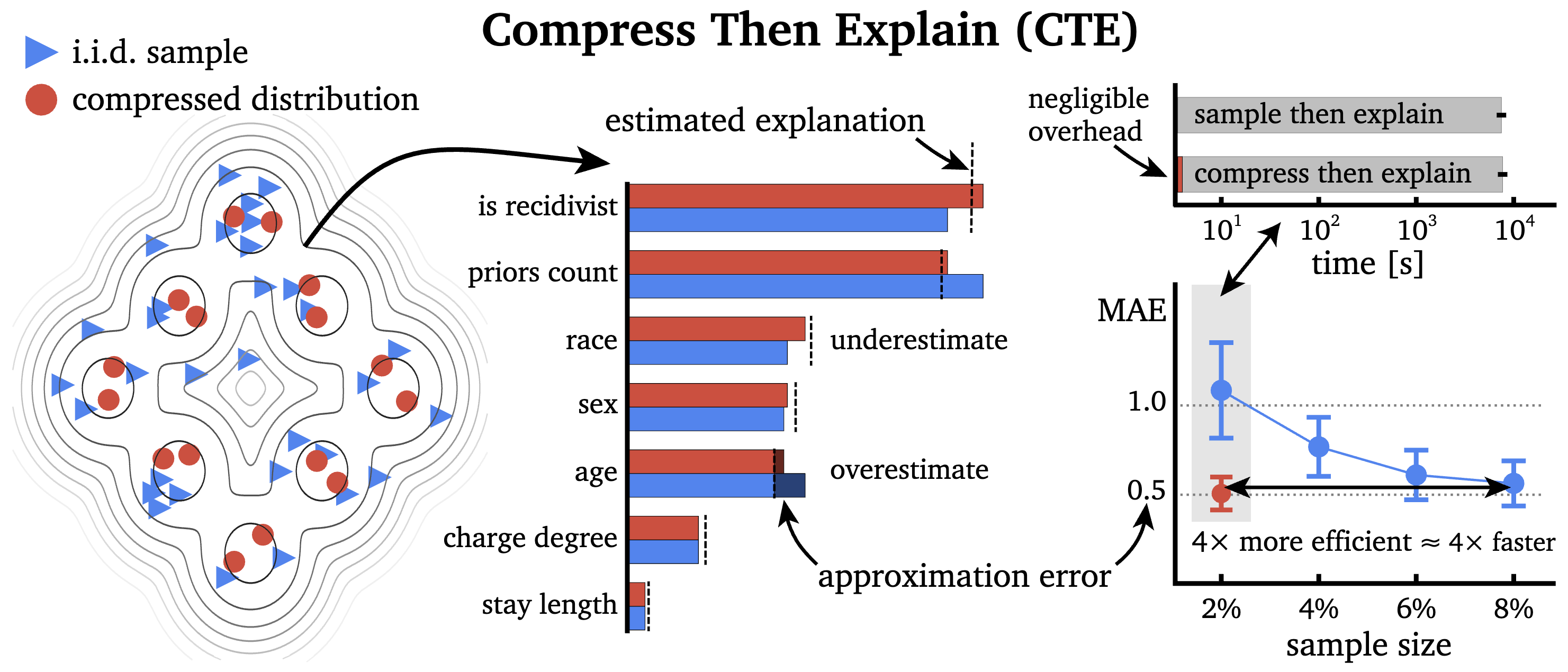
Hubert Baniecki, Giuseppe Casalicchio, Bernd Bischl, Przemyslaw Biecek
ICLR (2025)
We discover a connection between explanation estimation and distribution compression that significantly improves the approximation of feature attributions, importance, and effects.
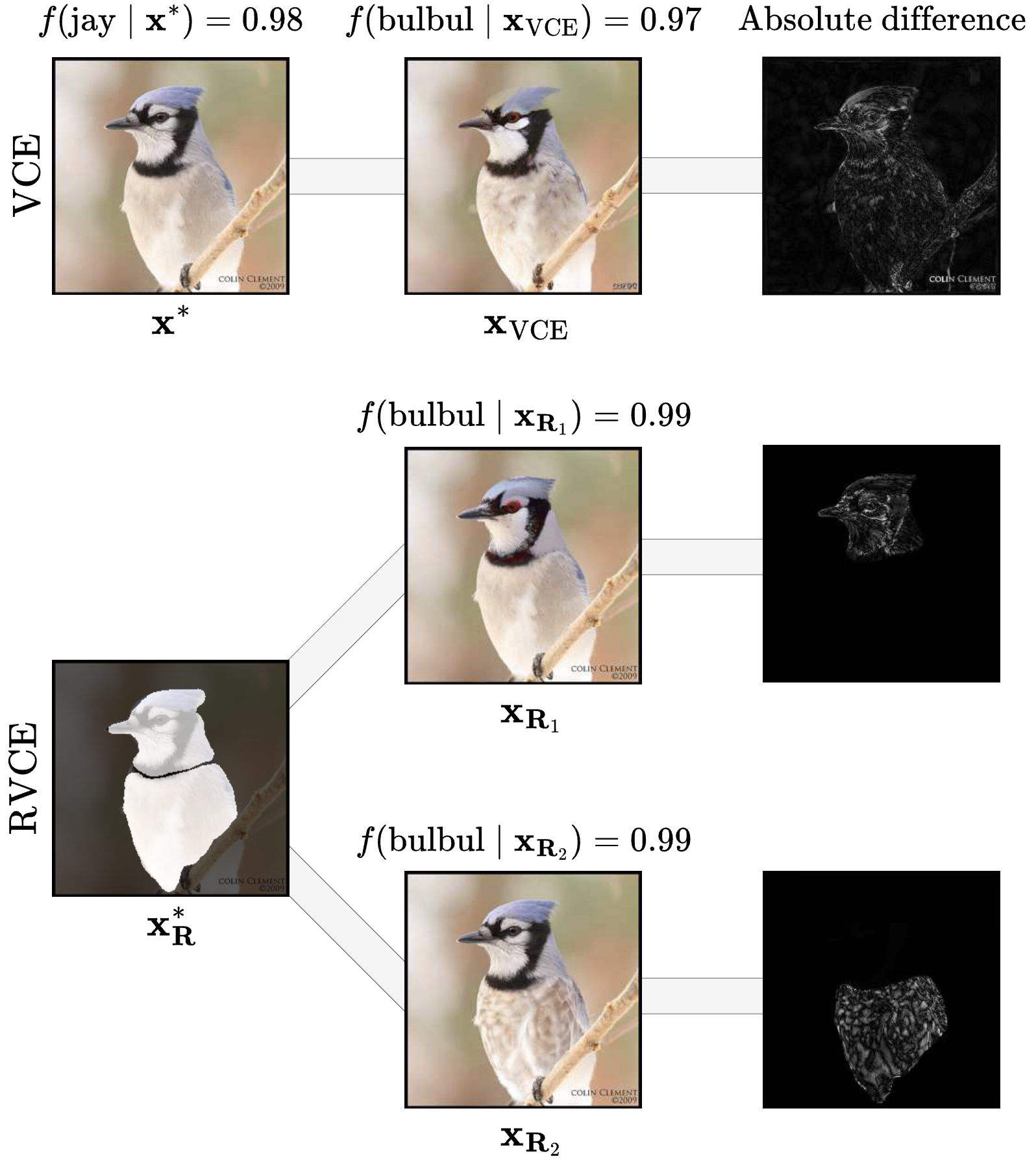
Bartlomiej Sobieski, Jakub Grzywaczewski, Bartlomiej Sadlej, Matthew Tivnan, Przemyslaw Biecek
ICLR (2025)
We propose a framework for visual counterfactual explanations with region constraint and develop an algorithm based on Image-to-Image Schrödinger Bridge that sets new state-of-the-art.
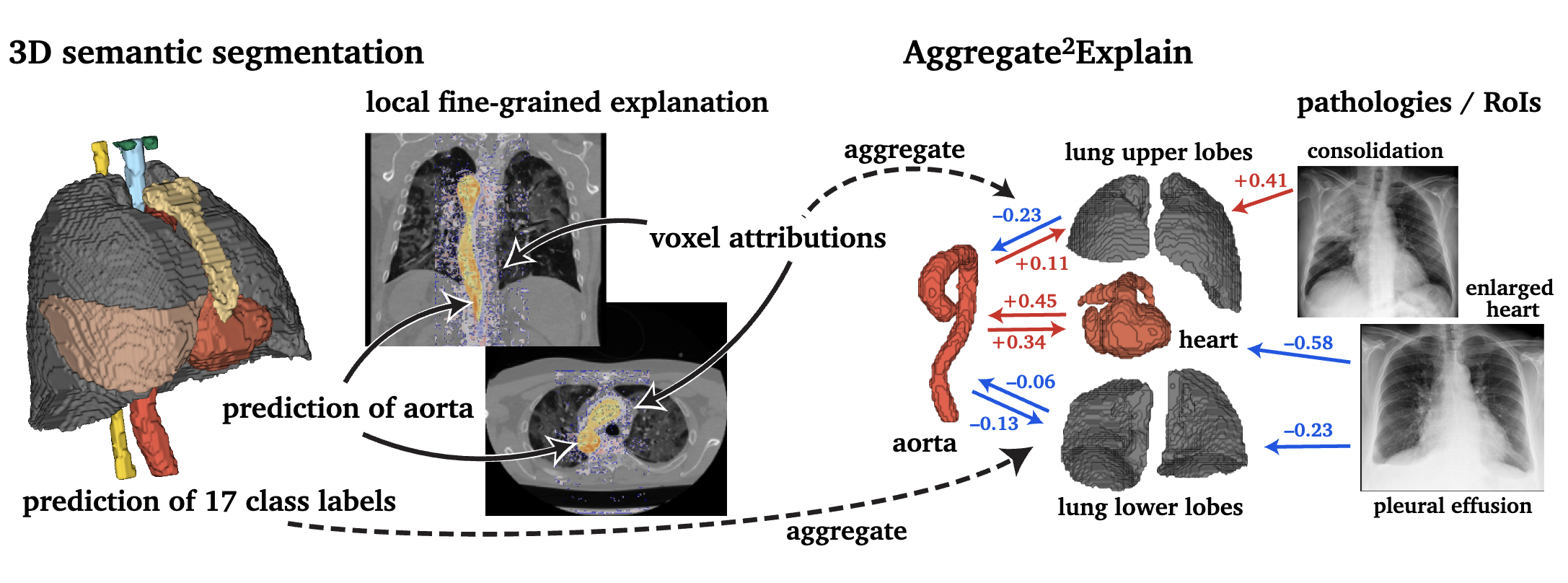
Maciej Chrabaszcz=, Hubert Baniecki=, Piotr Komorowski, Szymon Płotka, Przemysław Biecek
WACV (2025)
We introduce Agg^2Exp, a methodology for aggregating fine-grained voxel attributions of the segmentation model’s predictions. Unlike classical explanation methods that primarily focus on the local feature attribution, Agg^2Exp enables a more comprehensive global view on the importance of predicted segments in 3D images. As a concrete use-case, we apply Agg^2Exp to discover knowledge acquired by the Swin UNEt TRansformer model trained on the TotalSegmentator v2 dataset for segmenting anatomical structures in computed tomography medical images.
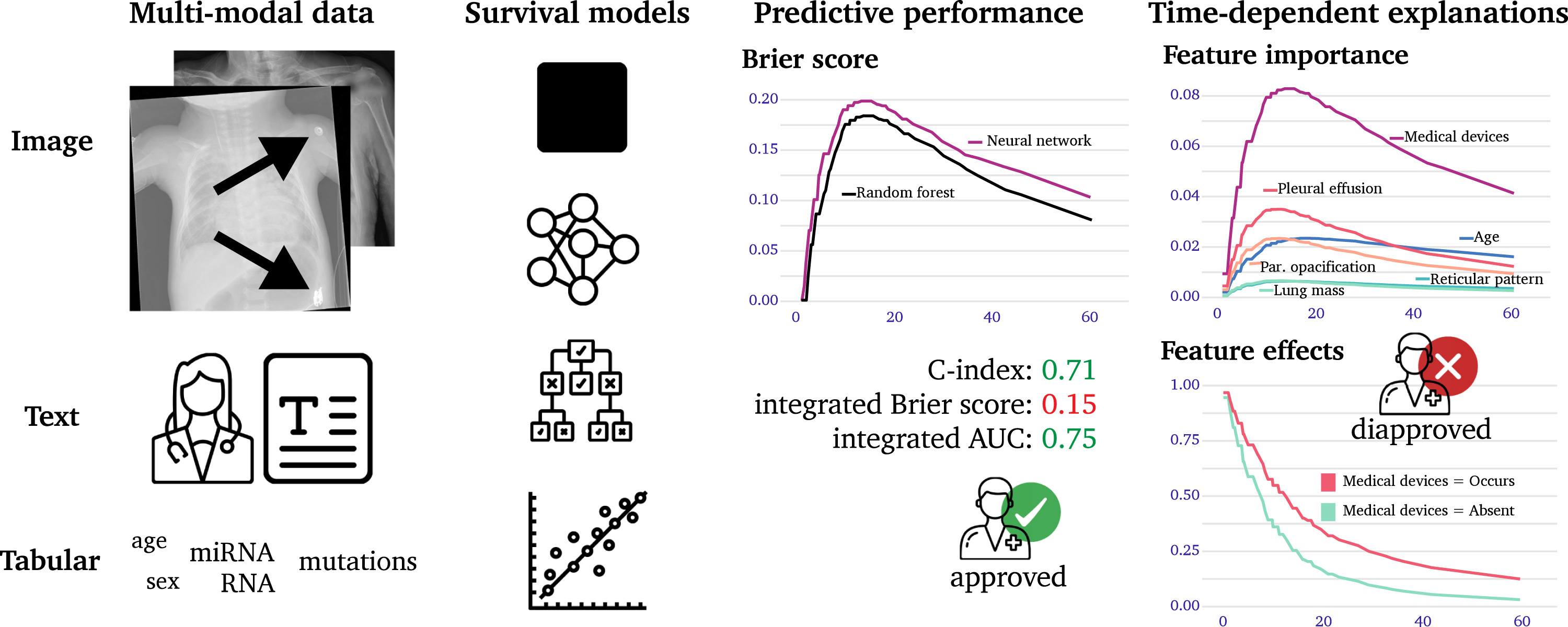
Hubert Baniecki, Bartlomiej Sobieski, Patryk Szatkowski, Przemyslaw Bombinski, Przemyslaw Biecek
Artificial Intelligence in Medicine (2025)
We formally introduce time-dependent feature effects and global feature importance explanations. We show how post-hoc interpretation methods allow for finding biases in AI systems predicting length of stay using a novel multi-modal dataset created from 1235 X-ray images with textual radiology reports annotated by human experts. Moreover, we evaluate cancer survival models beyond predictive performance to include the importance of multi-omics feature groups based on a large-scale benchmark comprising 11 datasets from The Cancer Genome Atlas (TCGA).
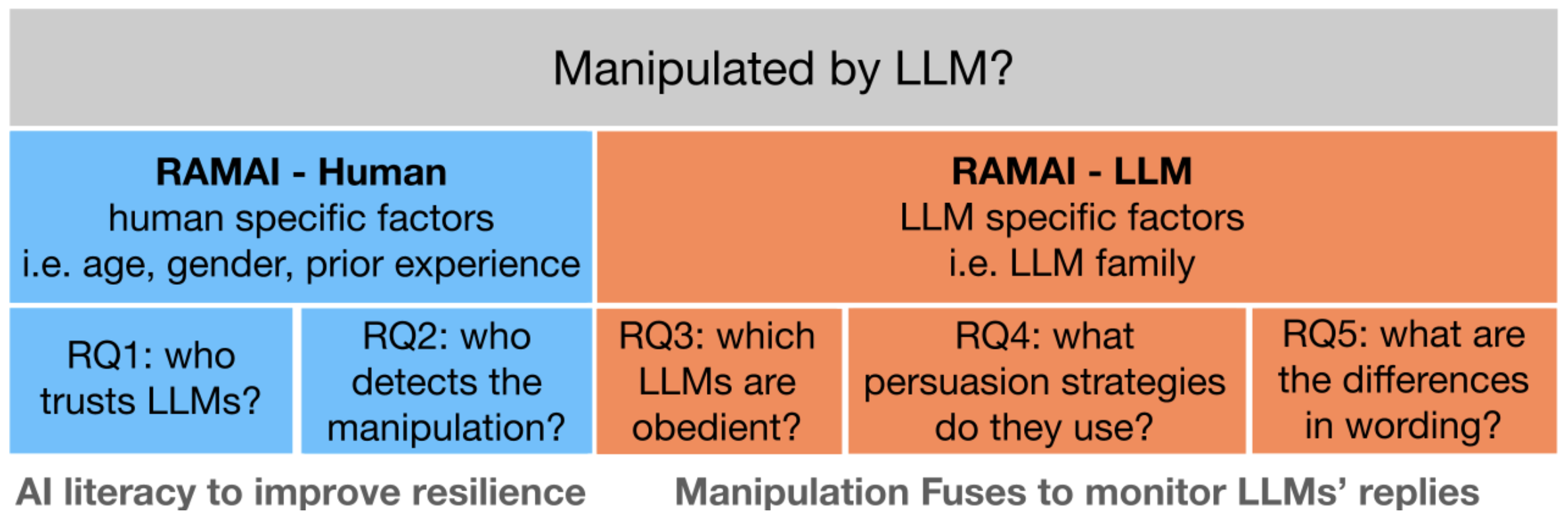
Piotr Wilczyński, Wiktoria Mieleszczenko-Kowszewicz, Przemysław Biecek
ECAI (2024)
We describe the results of two experiments designed to determine what characteristics of humans are associated with their susceptibility to LLM manipulation, and what characteristics of LLMs are associated with their manipulativeness potential. We explore human factors by conducting user studies in which participants answer general knowledge questions using LLM-generated hints, whereas LLM factors by provoking language models to create manipulative statements. In the long term, we put AI literacy at the forefront, arguing that educating society would minimize the risk of manipulation and its consequences.
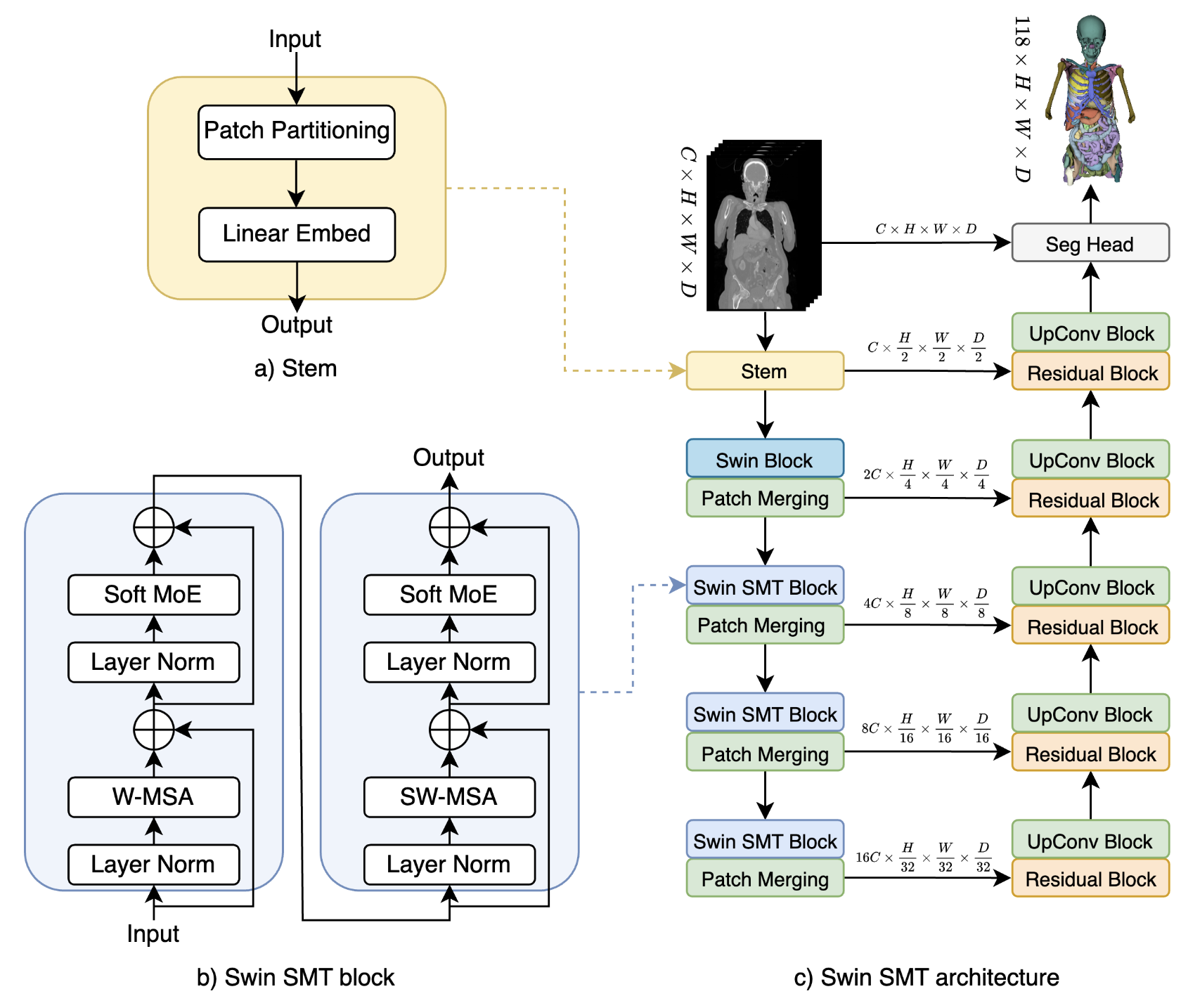
Szymon Płotka, Maciej Chrabaszcz, Przemyslaw Biecek
MICCAI (2024)
We introduce Swin Soft Mixture Transformer (Swin SMT), a novel architecture based on Swin UNETR, which incorporates a Soft Mixture-of-Experts (Soft MoE) to effectively handle complex and diverse long-range dependencies. The use of Soft MoE allows for scaling up model parameters maintaining a balance between computational complexity and segmentation performance in both training and inference modes. Comprehensive experimental results demonstrate that Swin SMT outperforms several state-of-the-art methods in 3D anatomical structure segmentation.
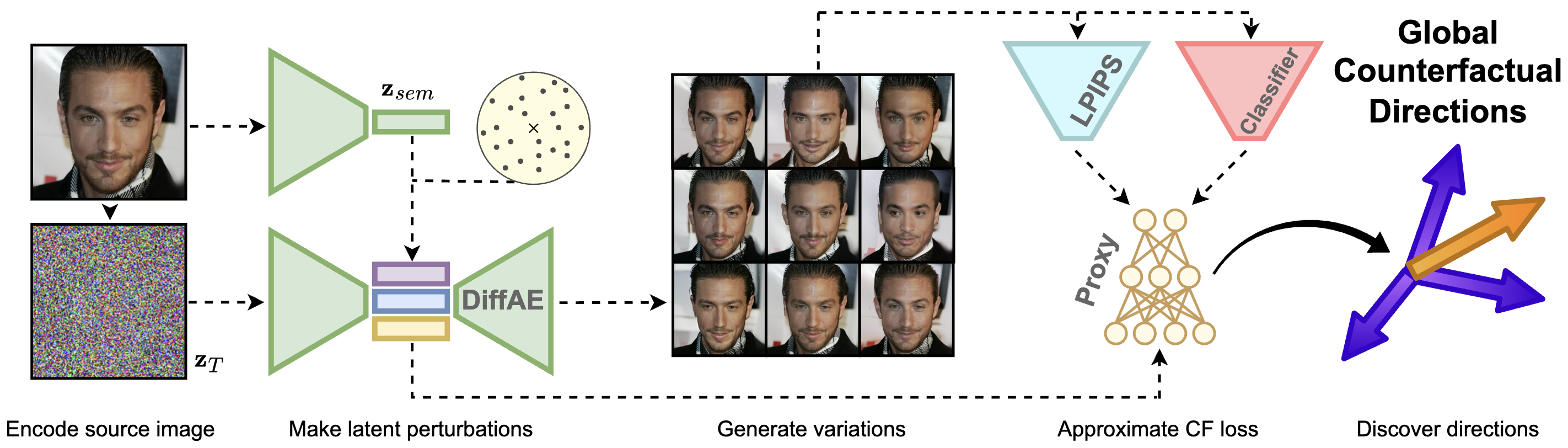
Bartlomiej Sobieski, Przemysław Biecek
ECCV (2024)
We discover that the latent space of Diffusion Autoencoders encodes the inference process of a given classifier in the form of global directions. We propose a novel proxy-based approach that discovers two types of these directions with the use of only single image in an entirely black-box manner. Precisely, g-directions allow for flipping the decision of a given classifier on an entire dataset of images, while h-directions further increase the diversity of explanations.
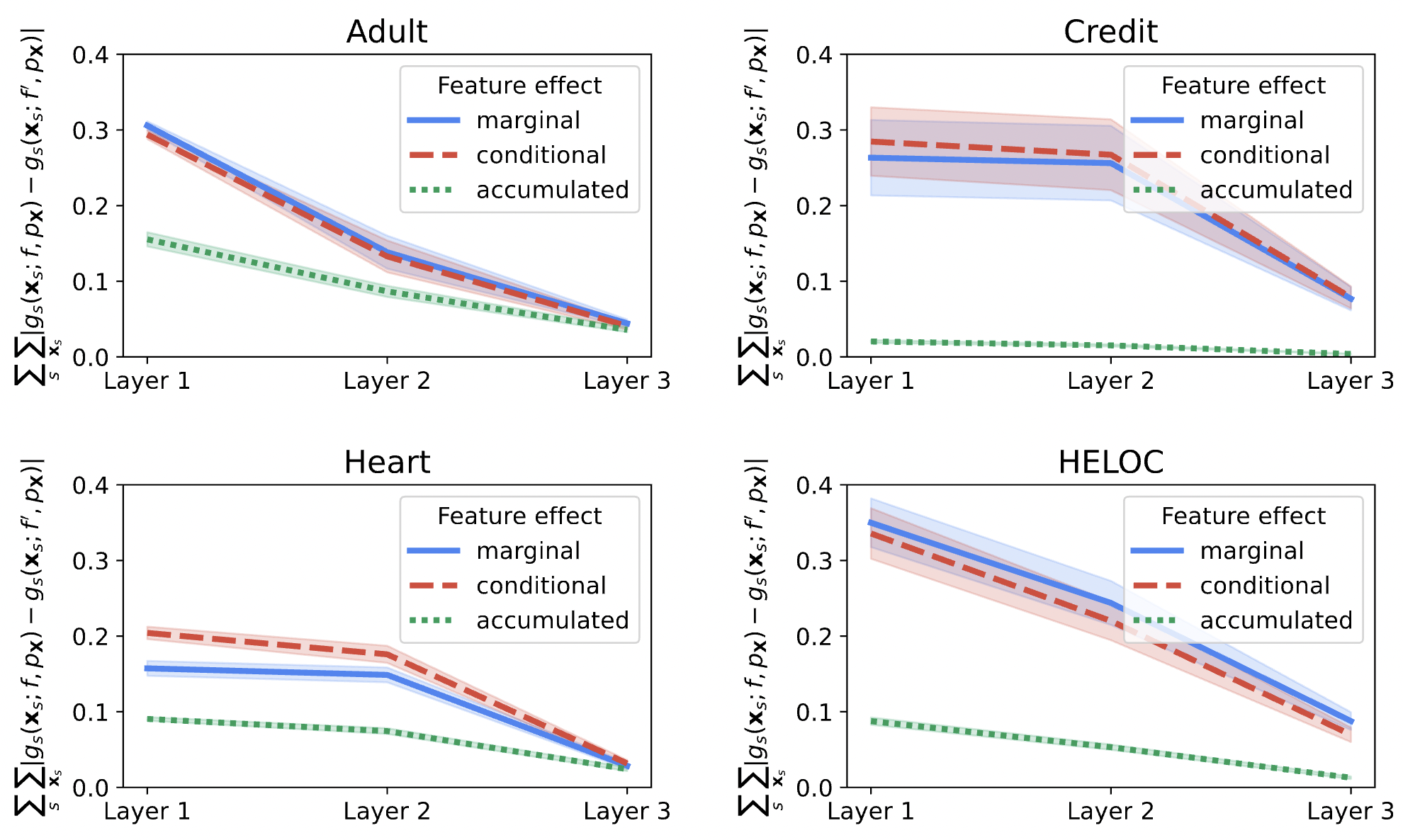
Hubert Baniecki, Giuseppe Casalicchio, Bernd Bischl, Przemyslaw Biecek
ECML PKDD (2024)
We introduce several theoretical bounds for evaluating the robustness of partial dependence plots and accumulated local effects. Our experimental results with synthetic and real-world datasets quantify the gap between the best and worst-case scenarios of (mis)interpreting machine learning predictions globally.
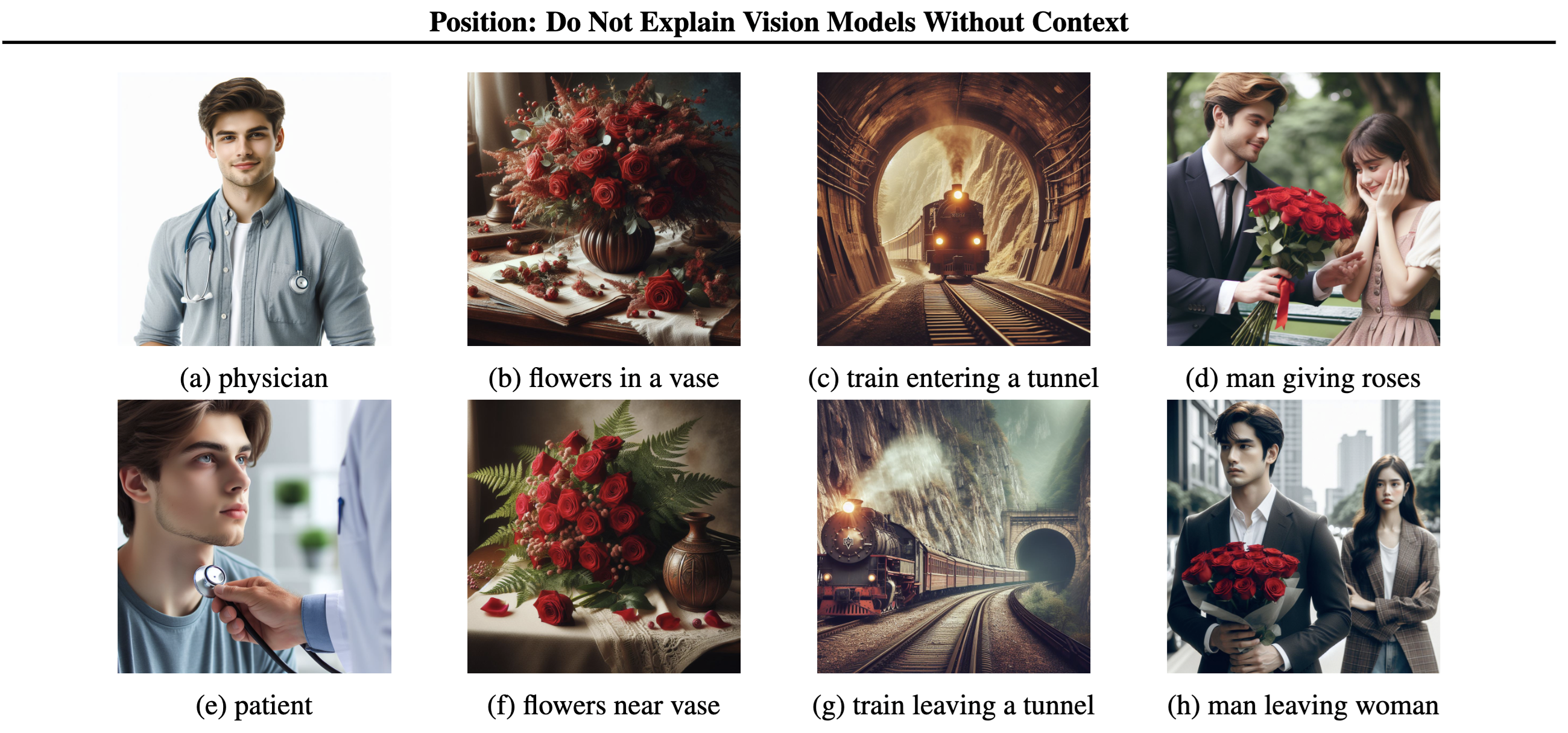
Paulina Tomaszewska, Przemysław Biecek
ICML (2024)
In this paper, we review the most popular methods of explaining computer vision models by pointing out that they do not take into account context information, propose new research directions that may lead to better use of context information in explaining computer vision models, and argue that a change in approach to explanations is needed from ‘where’ to ‘how’.
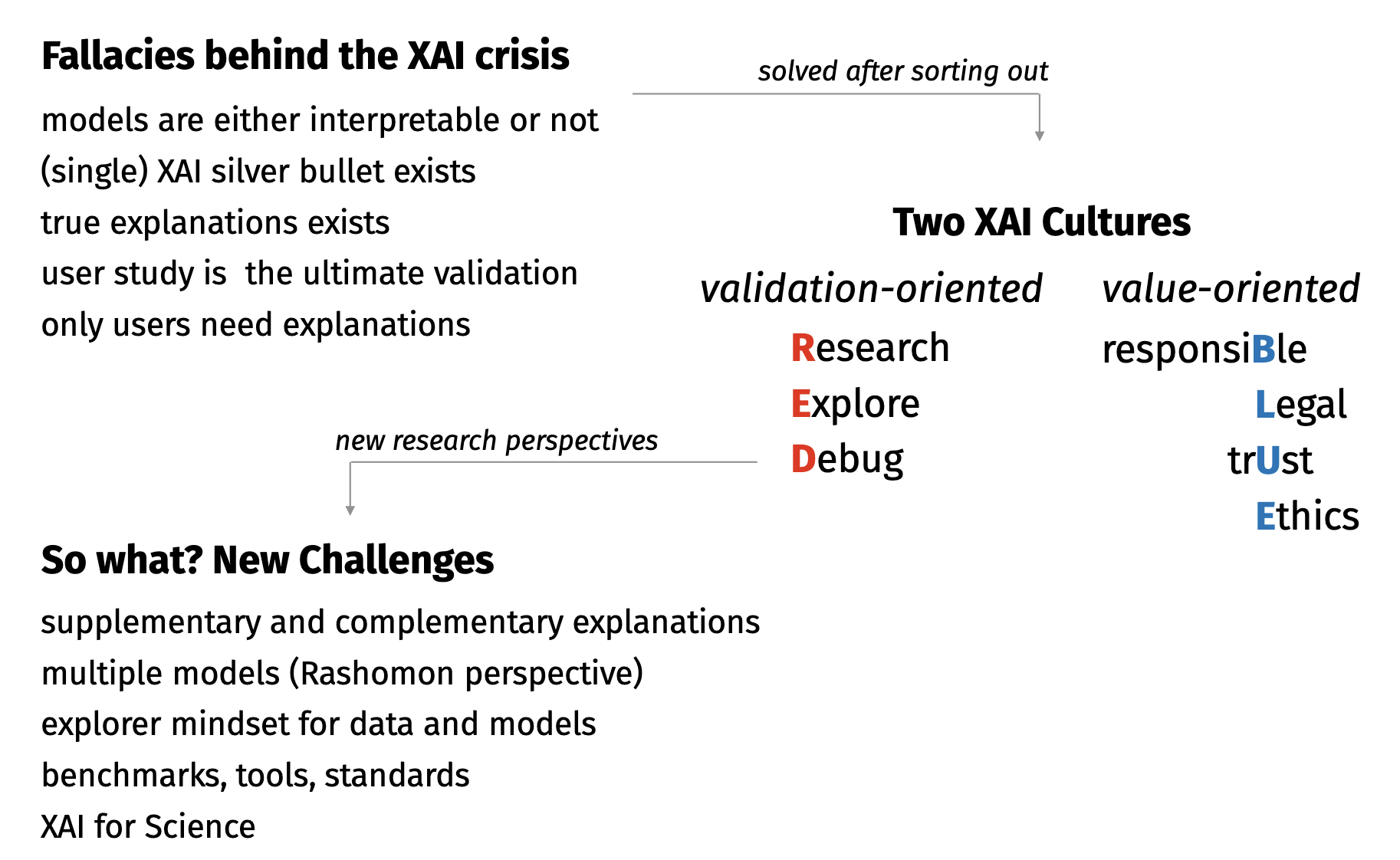
Przemysław Biecek, Wojciech Samek
ICML (2024)
Explainable Artificial Intelligence (XAI) is a young but very promising field of research. Unfortunately, the progress in this field is currently slowed down by divergent and incompatible goals. In this paper, we separate various threads tangled within the area of XAI into two complementary cultures of human/value-oriented explanations (BLUE XAI) and model/validation-oriented explanations (RED XAI).
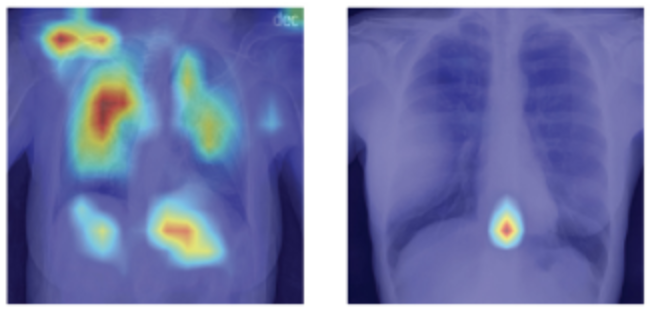
Oleksandr Davydko, Vladimir Pavlov, Przemysław Biecek, Luca Longo
XAI (2024)
A novel method (SRFAMap) is introduced to map the statistical radiomic feature attributions derived by applying the Integrated Gradients methods, often used in explainable AI, to image saliency maps. In detail, integrated gradients are used to compute radiomic feature attributions of chest X-ray scans for a ResNet-50 convolutional network model trained to distinguish healthy lungs from tuberculosis lesions. These are subsequently mapped to saliency maps over the original scans to facilitate their interpretation for diagnostics.
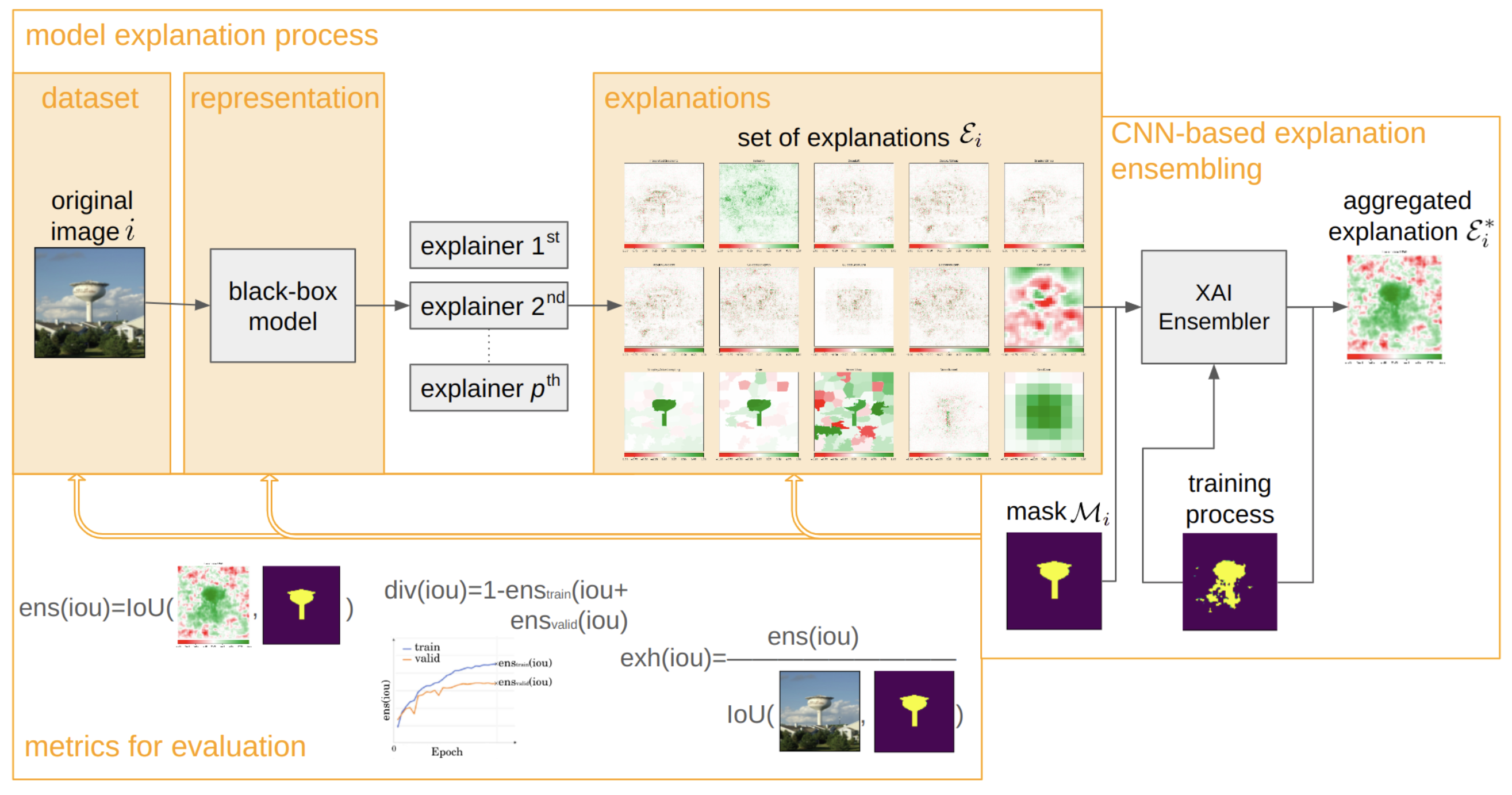
Weronika Hryniewska-Guzik, Luca Longo, Przemysław Biecek
XAI (2024)
In this research manuscript, we explore the potential of ensembling explanations generated by deep classification models using convolutional model. Through experimentation and analysis, we aim to investigate the implications of combining explanations to uncover a more coherent and reliable patterns of the model’s behavior, leading to the possibility of evaluating the representation learned by the model.
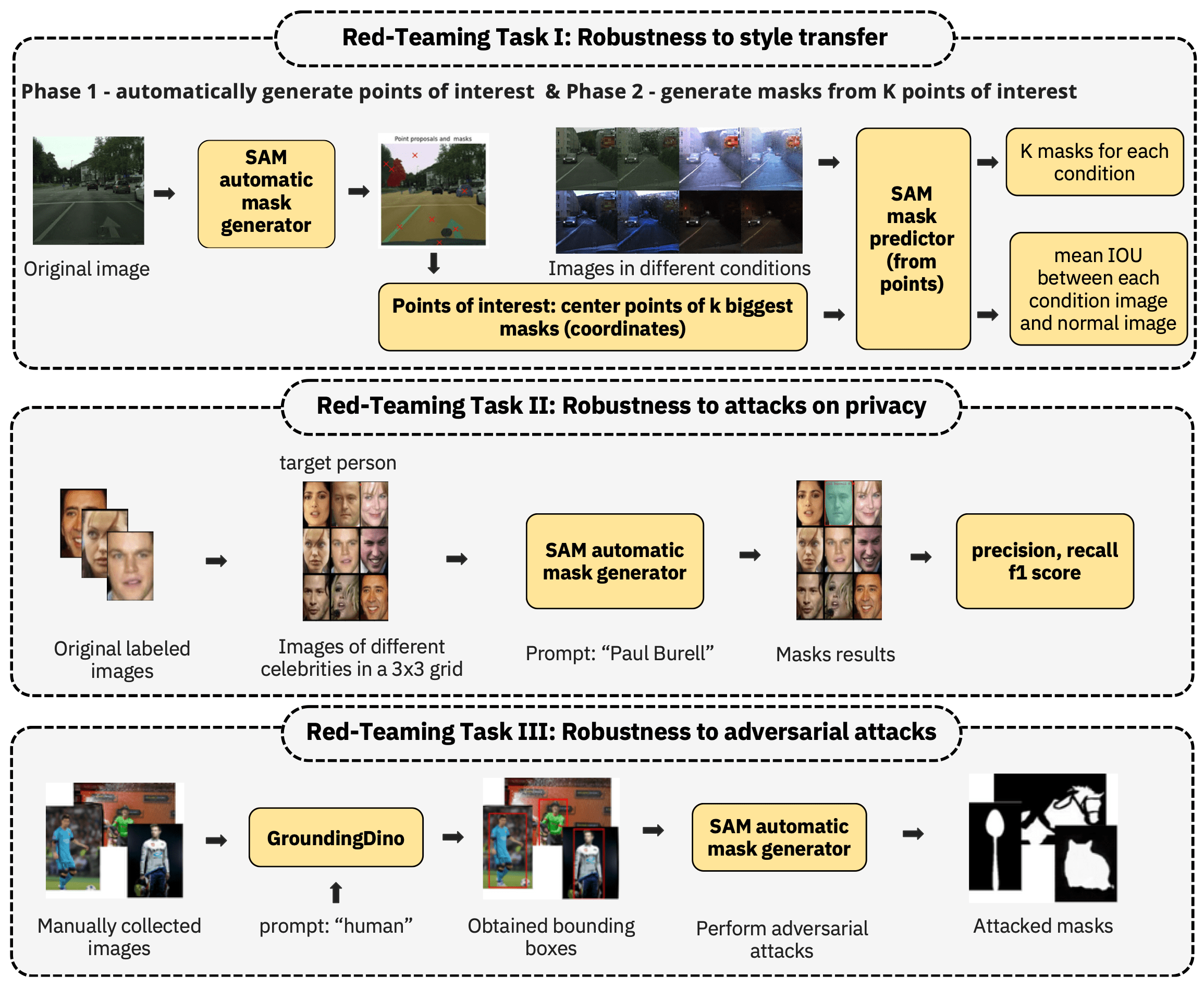
Krzysztof Jankowski=, Bartlomiej Sobieski=, Mateusz Kwiatkowski, Jakub Szulc, Michal Janik, Hubert Baniecki, Przemyslaw Biecek
CVPR Workshops (2024)
The Segment Anything Model is one of the first and most well-known foundation models for computer vision segmentation tasks. This work presents a multi-faceted red-teaming analysis of SAM. We analyze the impact of style transfer on segmentation masks. We assess whether the model can be used for attacks on privacy, such as recognizing celebrities’ faces. Finally, we check how robust the model is to adversarial attacks on segmentation masks under text prompts.
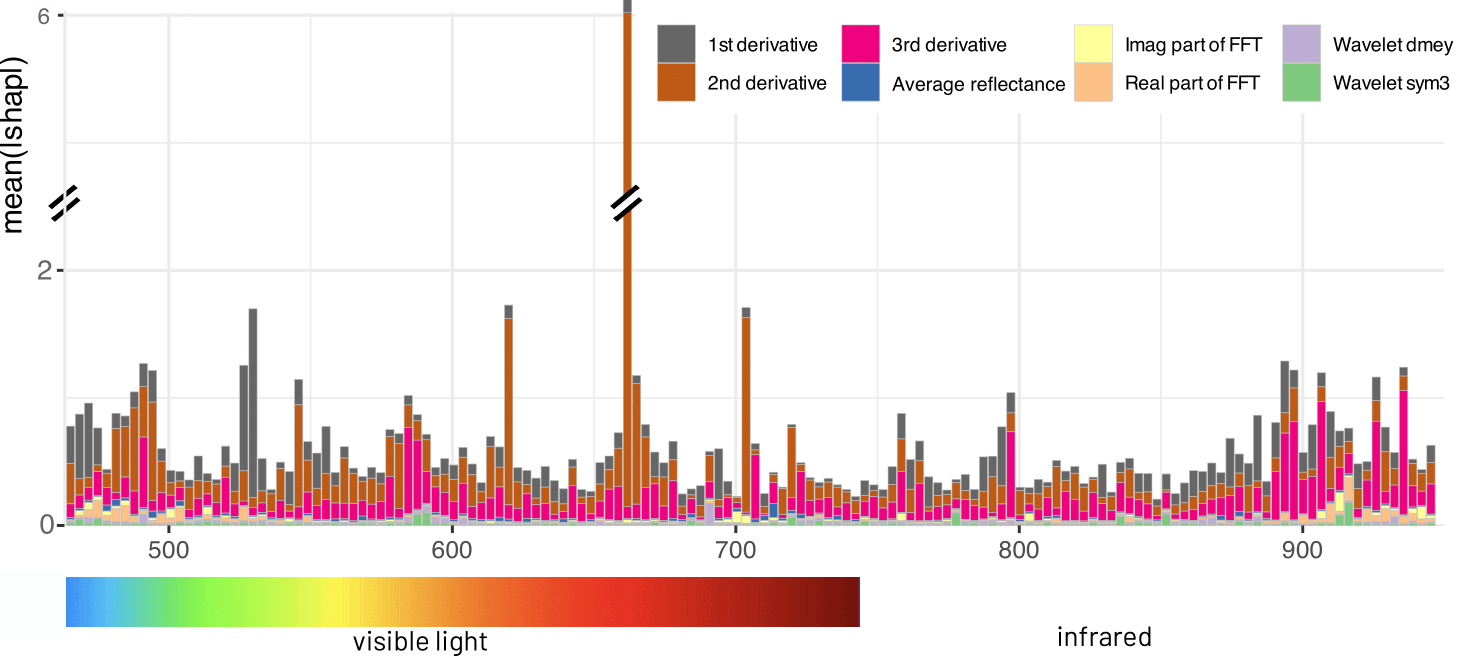
Vladimir Zaigrajew, Hubert Baniecki, Lukasz Tulczyjew, Agata M. Wijata, Jakub Nalepa, Nicolas Longépé, Przemyslaw Biecek
ICLR Workshops (2024)
Remote sensing applications require machine learning models that are reliable and robust, highlighting the importance of red teaming for uncovering flaws and biases. We introduce a novel red teaming approach for hyperspectral image analysis, specifically for soil parameter estimation in the Hyperview challenge. Utilizing SHAP for red teaming, we enhanced the top-performing model based on our findings. Additionally, we introduced a new visualization technique to improve model understanding in the hyperspectral domain.
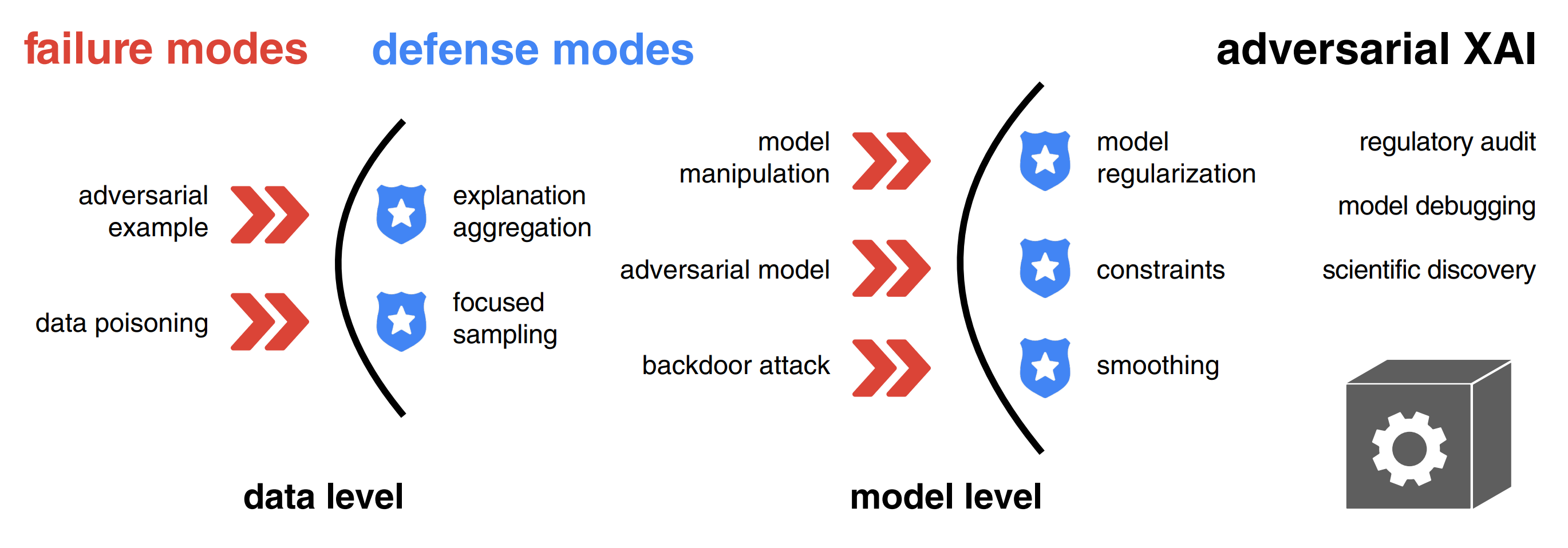
Hubert Baniecki, Przemysław Biecek
Information Fusion (2024)
Explanations of machine learning models can be manipulated. We introduce a unified notation and taxonomy of adversarial attacks on explanations. Adversarial examples, data poisoning, and backdoor attacks are key safety issues in XAI. Defense methods like model regularization improve the robustness of explanations. We outline the emerging research directions in adversarial XAI.
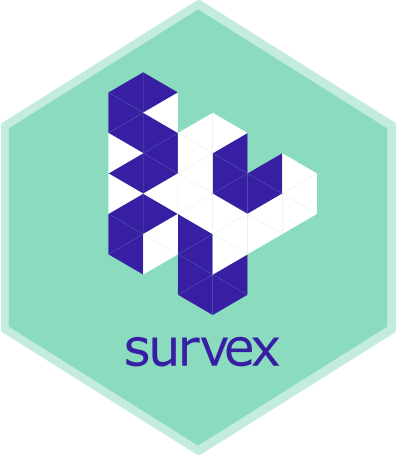
Mikołaj Spytek, Mateusz Krzyziński, Sophie Hanna Langbein, Hubert Baniecki, Marvin N Wright, Przemysław Biecek
Bioinformatics (2023)
This paper demonstrates the functionalities of the survex package, which provides a comprehensive set of tools for explaining machine learning survival models. The capabilities of the proposed software encompass understanding and diagnosing survival models, which can lead to their improvement. By revealing insights into the decision-making process, such as variable effects and importances, survex enables the assessment of model reliability and the detection of biases. Thus, promoting transparency and responsibility in sensitive areas.
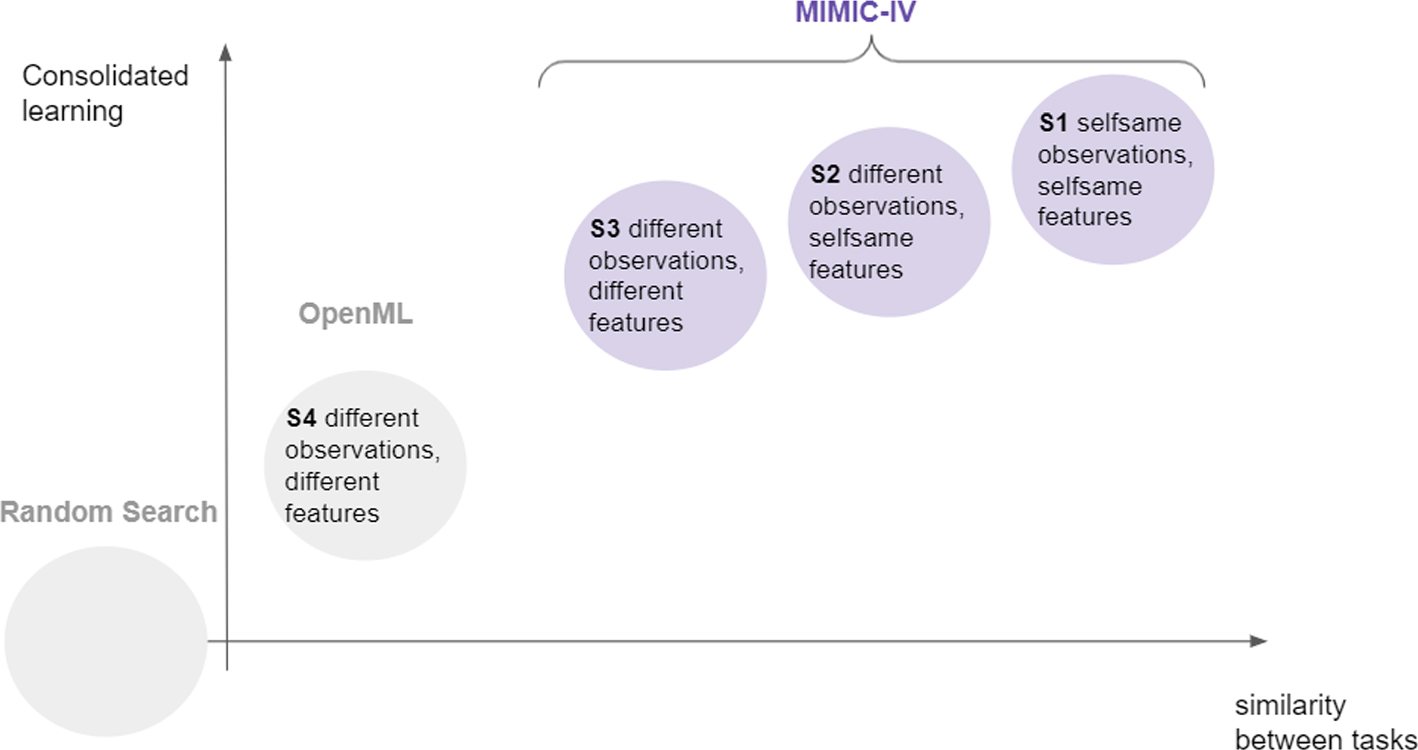
Katarzyna Woźnica, Mateusz Grzyb, Zuzanna Trafas, Przemysław Biecek
Machine Learning (2023)
This paper proposes a new formulation of the tuning problem, called consolidated learning, more suited to practical challenges faced by model developers, in which a large number of predictive models are created on similar datasets. We show that a carefully selected static portfolio of hyperparameter configurations yields good results for anytime optimization, while maintaining the ease of use and implementation. We demonstrate the effectiveness of this approach through an empirical study for the XGBoost algorithm and the newly created metaMIMIC benchmarks of predictive tasks extracted from the MIMIC-IV medical database.
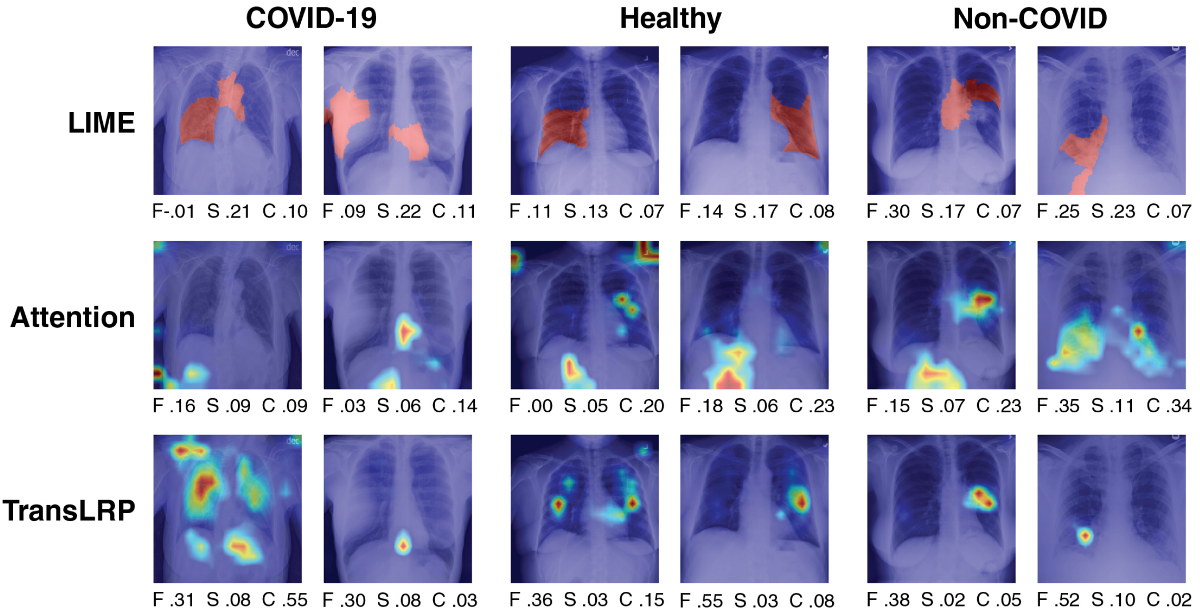
Piotr Komorowski, Hubert Baniecki, Przemysław Biecek
CVPR Workshop on Explainable AI for Computer Vision (2023)
This paper investigates the performance of various interpretation methods on a Vision Transformer (ViT) applied to classify chest X-ray images. We introduce the notion of evaluating faithfulness, sensitivity, and complexity of ViT explanations. The obtained results indicate that Layerwise relevance propagation for transformers outperforms Local interpretable model-agnostic explanations and Attention visualization, providing a more accurate and reliable representation of what a ViT has actually learned.
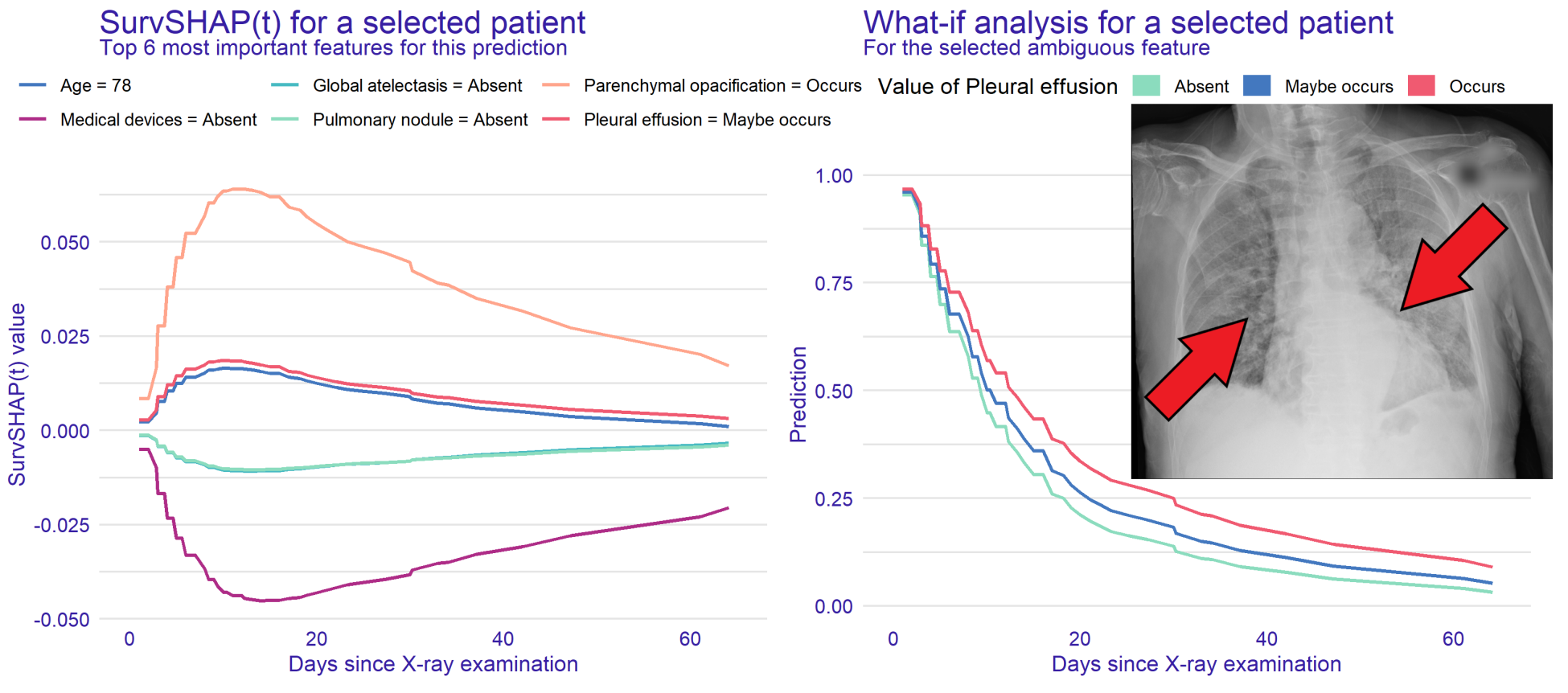
Hubert Baniecki, Bartlomiej Sobieski, Przemysław Bombiński, Patryk Szatkowski, Przemysław Biecek
International Conference on Artificial Intelligence in Medicine (2023)
To what extent can the patient’s length of stay in a hospital be predicted using only an X-ray image? We answer this question by comparing the performance of machine learning survival models on a novel multi-modal dataset created from 1235 images with textual radiology reports annotated by humans. We introduce time-dependent model explanations into the human-AI decision making process. For reproducibility, we open-source code and the TLOS dataset at this URL.
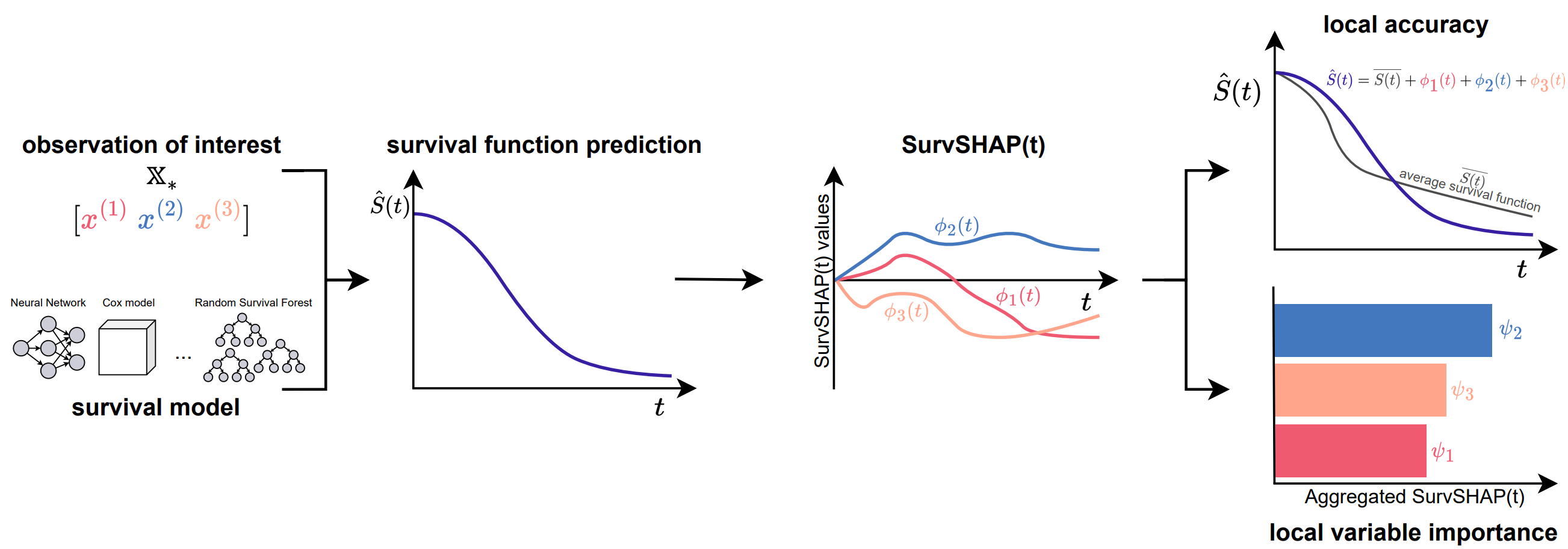
Mateusz Krzyziński, Mikołaj Spytek, Hubert Baniecki, Przemysław Biecek
Knowledge-Based Systems (2023)
In this paper, we introduce SurvSHAP(t), the first time-dependent explanation that allows for interpreting survival black-box models. The proposed methods aim to enhance precision diagnostics and support domain experts in making decisions. SurvSHAP(t) is model-agnostic and can be applied to all models with functional output. We provide an accessible implementation of time-dependent explanations in Python at this URL.
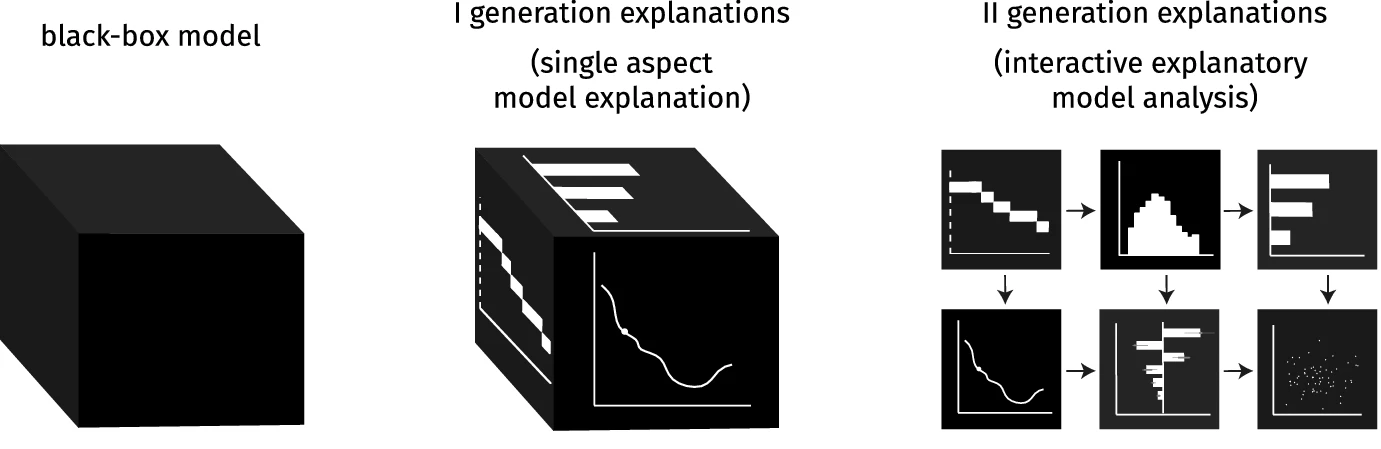
Hubert Baniecki, Dariusz Parzych, Przemyslaw Biecek
Data Mining and Knowledge Discovery (2023)
This paper proposes how different Explanatory Model Analysis (EMA) methods complement each other and discusses why it is essential to juxtapose them. The introduced process of Interactive EMA (IEMA) derives from the algorithmic side of explainable machine learning and aims to embrace ideas developed in cognitive sciences. We formalize the grammar of IEMA to describe human-model interaction. We conduct a user study to evaluate the usefulness of IEMA, which indicates that an interactive sequential analysis of a model may increase the accuracy and confidence of human decision making.
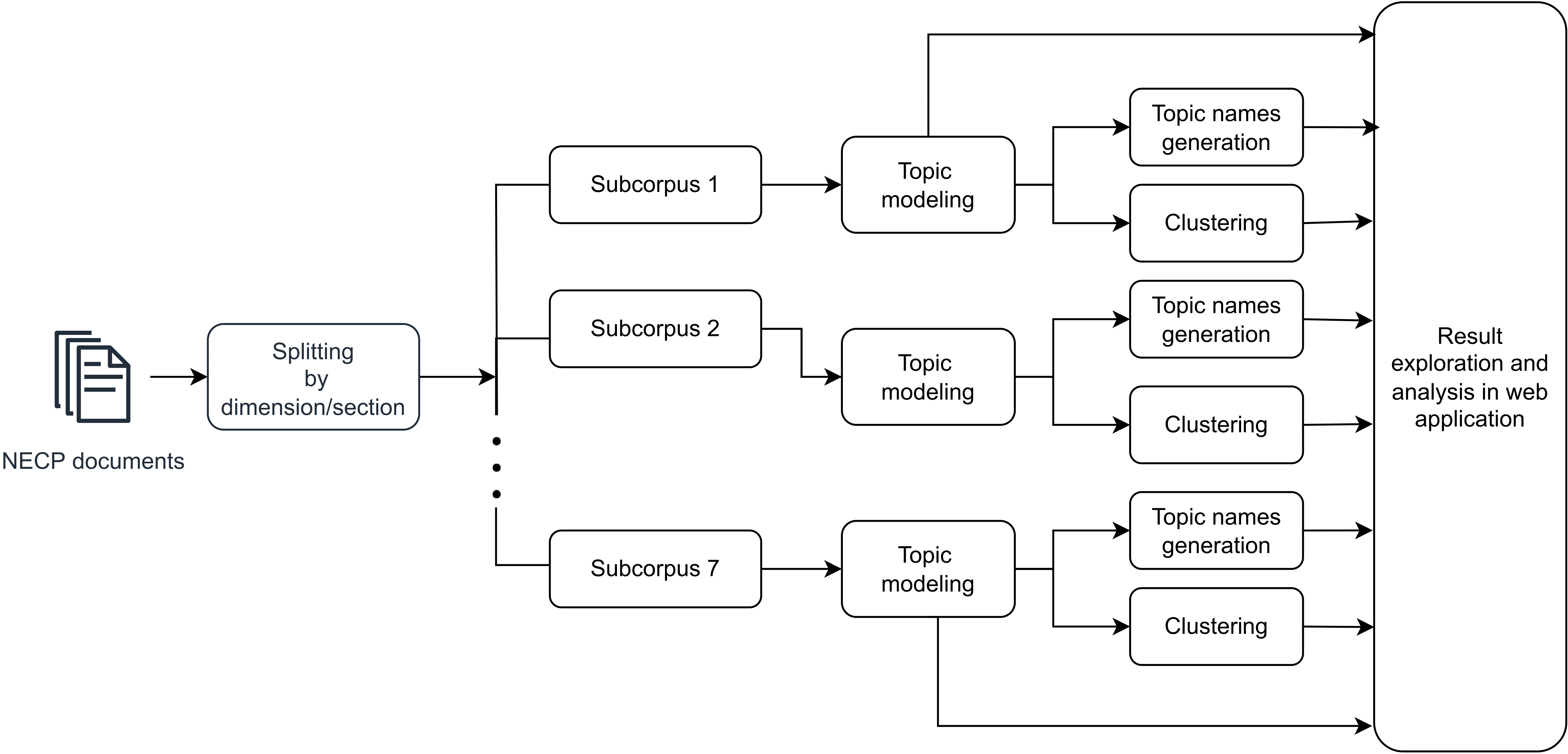
Artur Żółkowski, Mateusz Krzyziński, Piotr Wilczyński, Stanisław Giziński, Emilia Wiśnios, Bartosz Pieliński, Julian Sienkiewicz, Przemysław Biecek
NeurIPS Workshop on Tackling Climate Change with Machine Learning (2022)
In this work, we use a Latent Dirichlet Allocation-based pipeline for the automatic summarization and analysis of 10-years of national energy and climate plans (NECPs) for the period from 2021 to 2030, established by 27 Member States of the European Union. We focus on analyzing policy framing, the language used to describe specific issues, to detect essential nuances in the way governments frame their climate policies and achieve climate goals.
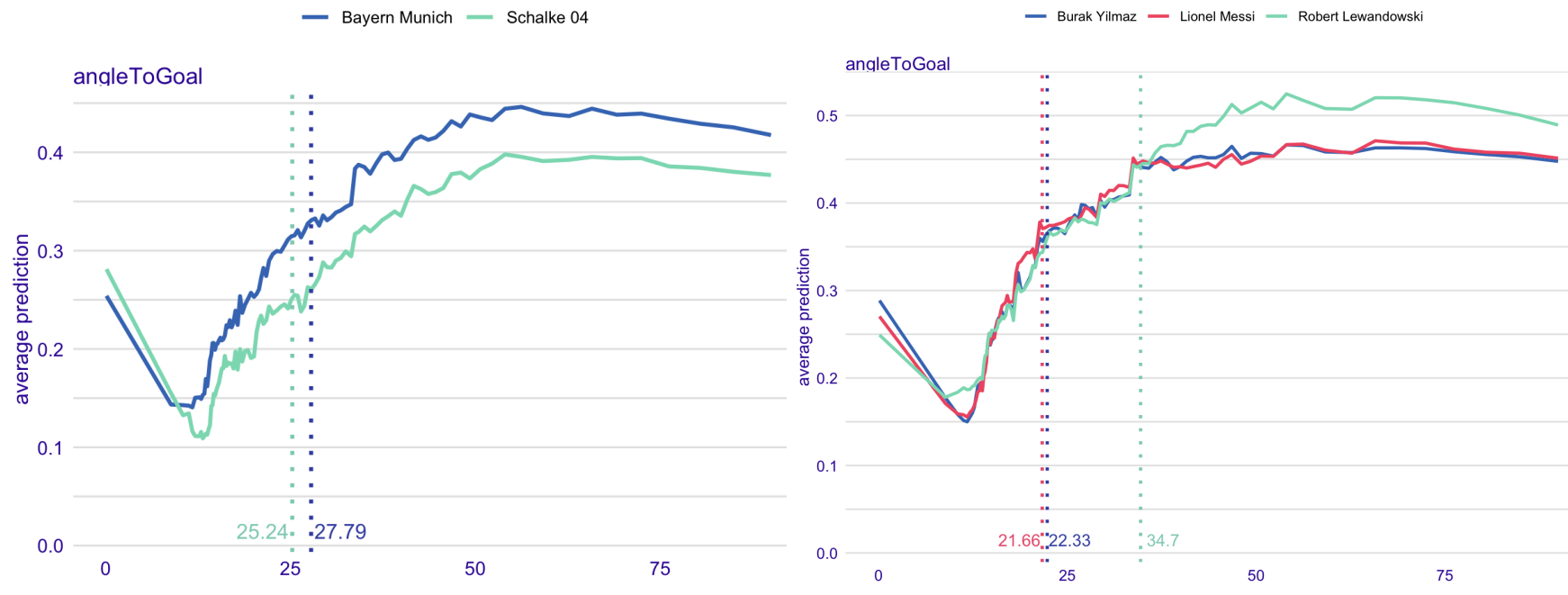
Mustafa Cavus, Przemyslaw Biecek
International Conference on Data Science and Advanced Analytics (2022)
The expected goal provides a more representative measure of the team and player performance which also suit the low-scoring nature of football instead of the score in modern football. This paper proposes an accurate expected goal model trained on 315,430 shots from seven seasons between 2014-15 and 2020-21 of the top-five European football leagues. Moreover, we demonstrate a practical application of aggregated profiles to explain a group of observations on an accurate expected goal model for monitoring the team and player performance.
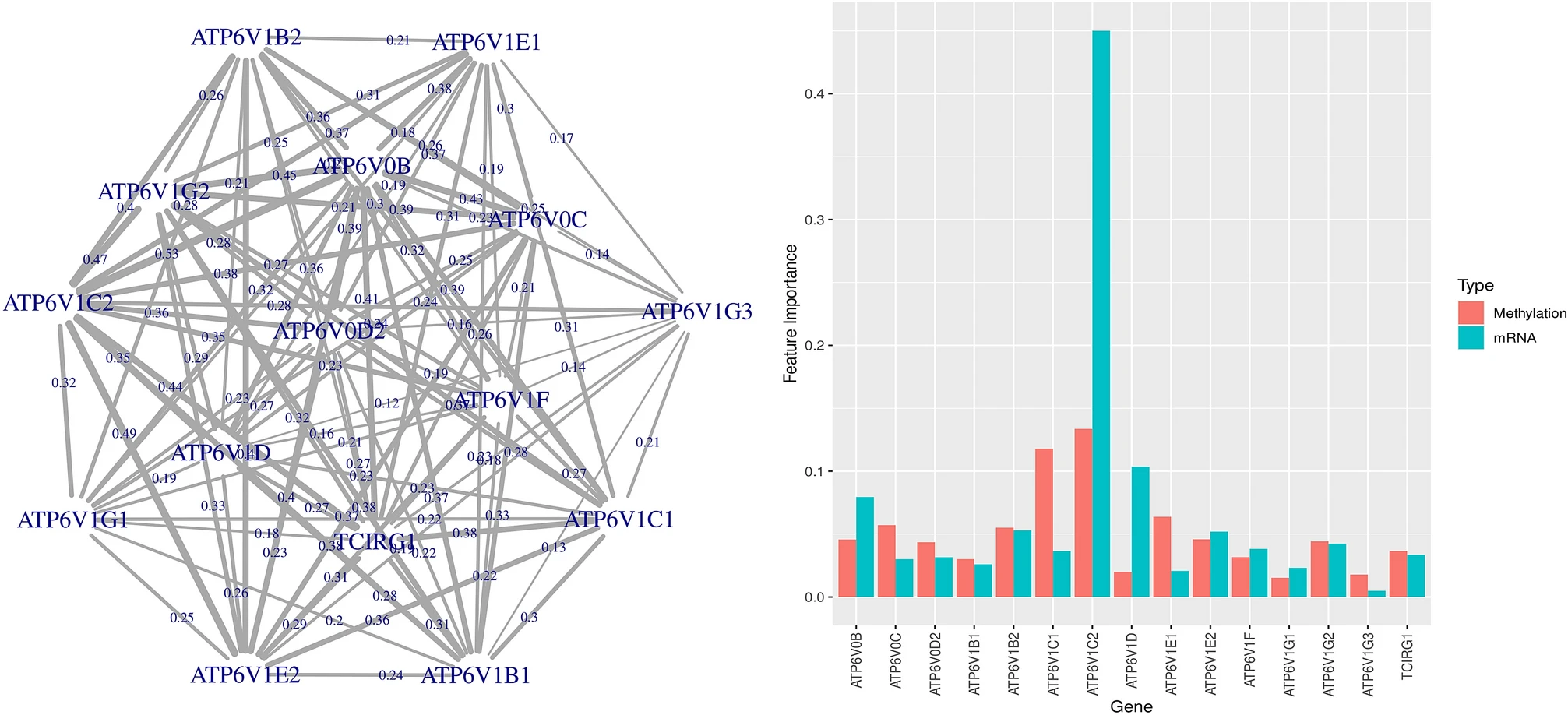
Bastian Pfeifer, Hubert Baniecki, Anna Saranti, Przemyslaw Biecek, Andreas Holzinger
Scientific Reports (2022)
In this work, we demonstrate subnetwork detection based on multi-modal node features using a novel Greedy Decision Forest (GDF) with inherent interpretability. The latter will be a crucial factor to retain experts and gain their trust in such algorithms. To demonstrate a concrete application example, we focus on bioinformatics, systems biology and particularly biomedicine, but the presented methodology is applicable in many other domains as well. Our proposed explainable approach can help to uncover disease-causing network modules from multi-omics data to better understand complex diseases such as cancer.
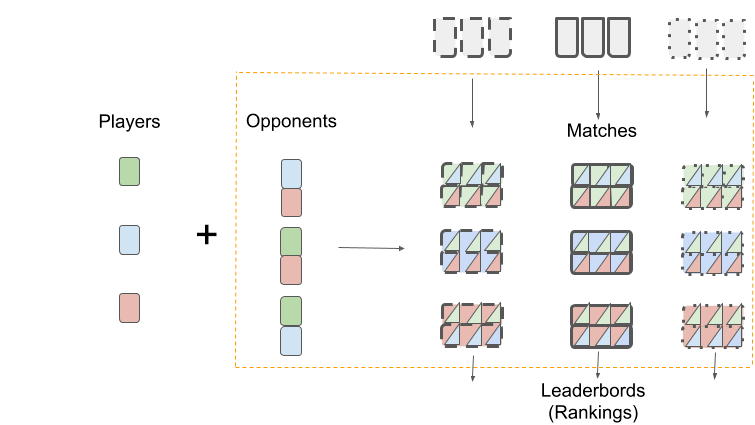
Alicja Gosiewska, Katarzyna Woźnica, Przemysław Biecek
Nature Machine Intelligence (2022)
Elo-based predictive power (EPP) meta-score that is built on other performance measures and allows for interpretable comparisons of models. Differences between this score have a probabilistic interpretation and can be compared directly between data sets. Furthermore, this meta-score allows for an assessment of ranking fitness. We prove the properties of the Elo-based predictive power meta-score and support them with empirical results on a large-scale benchmark of 30 classification data sets. Additionally, we propose a unified benchmark ontology that provides a uniform description of benchmarks.
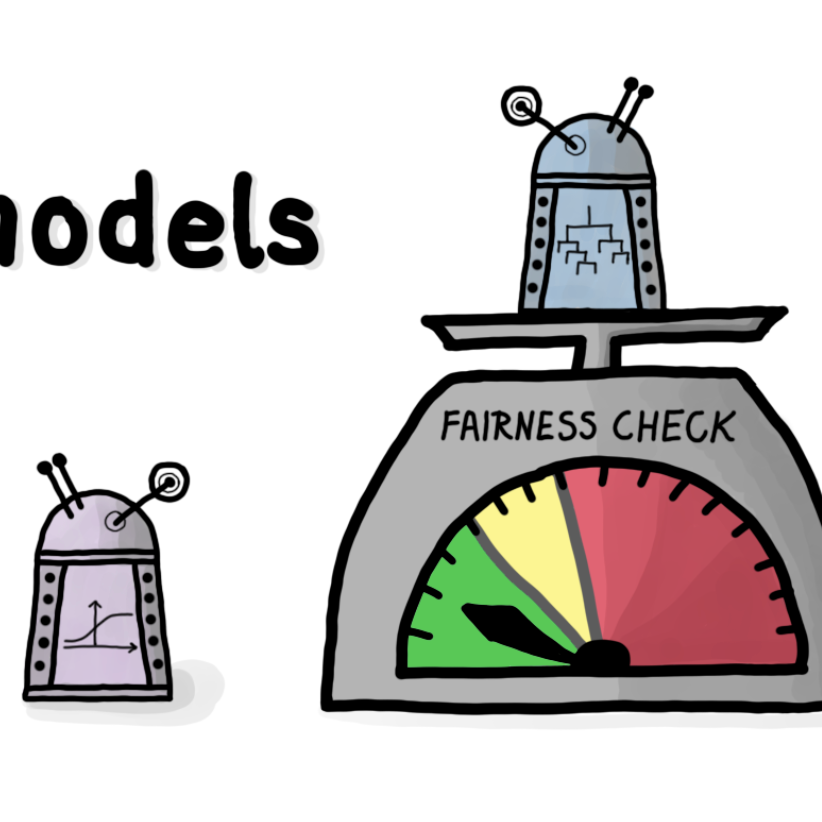
Jakub Wiśniewski, Przemyslaw Biecek
The R Journal (2022)
This article introduces an R package fairmodels that helps to validate fairness and eliminate bias in binary classification models quickly and flexibly. It offers a model-agnostic approach to bias detection, visualization, and mitigation. The implemented functions and fairness metrics enable model fairness validation from different perspectives. In addition, the package includes a series of methods for bias mitigation that aim to diminish the discrimination in the model. The package is designed to examine a single model and facilitate comparisons between multiple models.
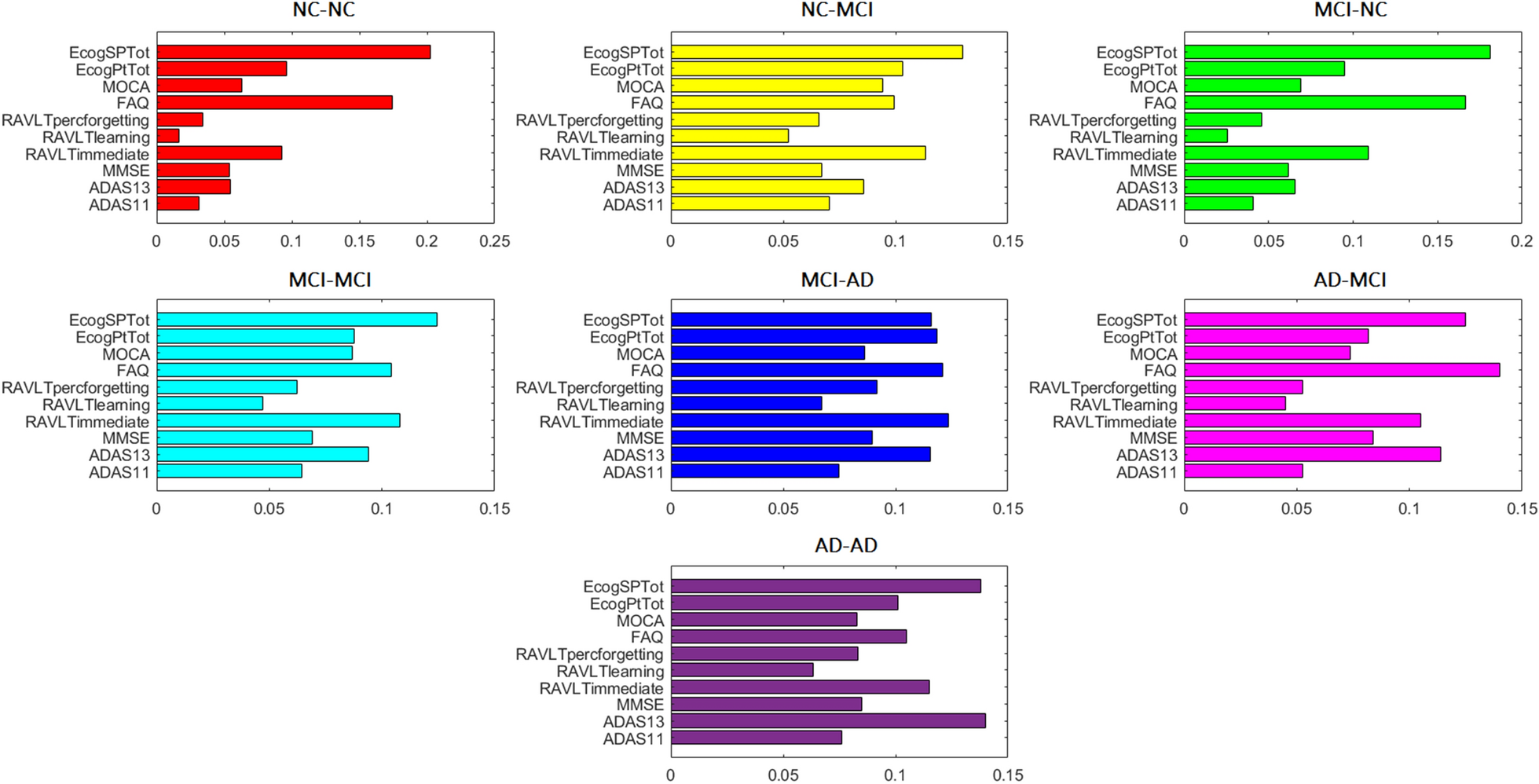
Angela Lombardi, Domenico Diacono, Nicola Amoroso, Przemysław Biecek, Alfonso Monaco, Loredana Bellantuono, Ester Pantaleo, Giancarlo Logroscino, Roberto De Blasi, Sabina Tangaro, Roberto Bellotti
Brain Informatics (2022)
In this work, we present a robust framework to (i) perform a threefold classification between healthy control subjects, individuals with cognitive impairment, and subjects with dementia using different cognitive indexes and (ii) analyze the variability of the explainability SHAP values associated with the decisions taken by the predictive models. We demonstrate that the SHAP values can accurately characterize how each index affects a patient’s cognitive status. Furthermore, we show that a longitudinal analysis of SHAP values can provide effective information on Alzheimer’s disease progression.
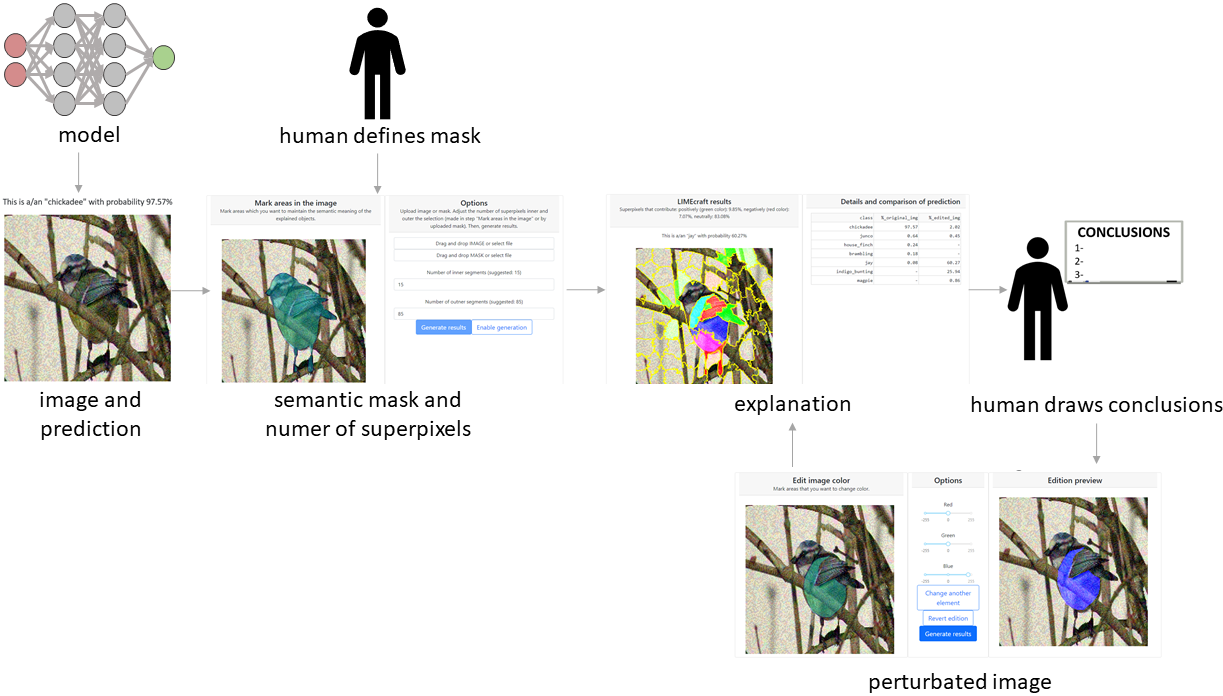
Weronika Hryniewska, Adrianna Grudzień, Przemysław Biecek
Machine Learning (2022)
LIMEcraft enhances the process of explanation by allowing a user to interactively select semantically consistent areas and thoroughly examine the prediction for the image instance in case of many image features. Experiments on several models show that our tool improves model safety by inspecting model fairness for image pieces that may indicate model bias. The code is available at: this URL.
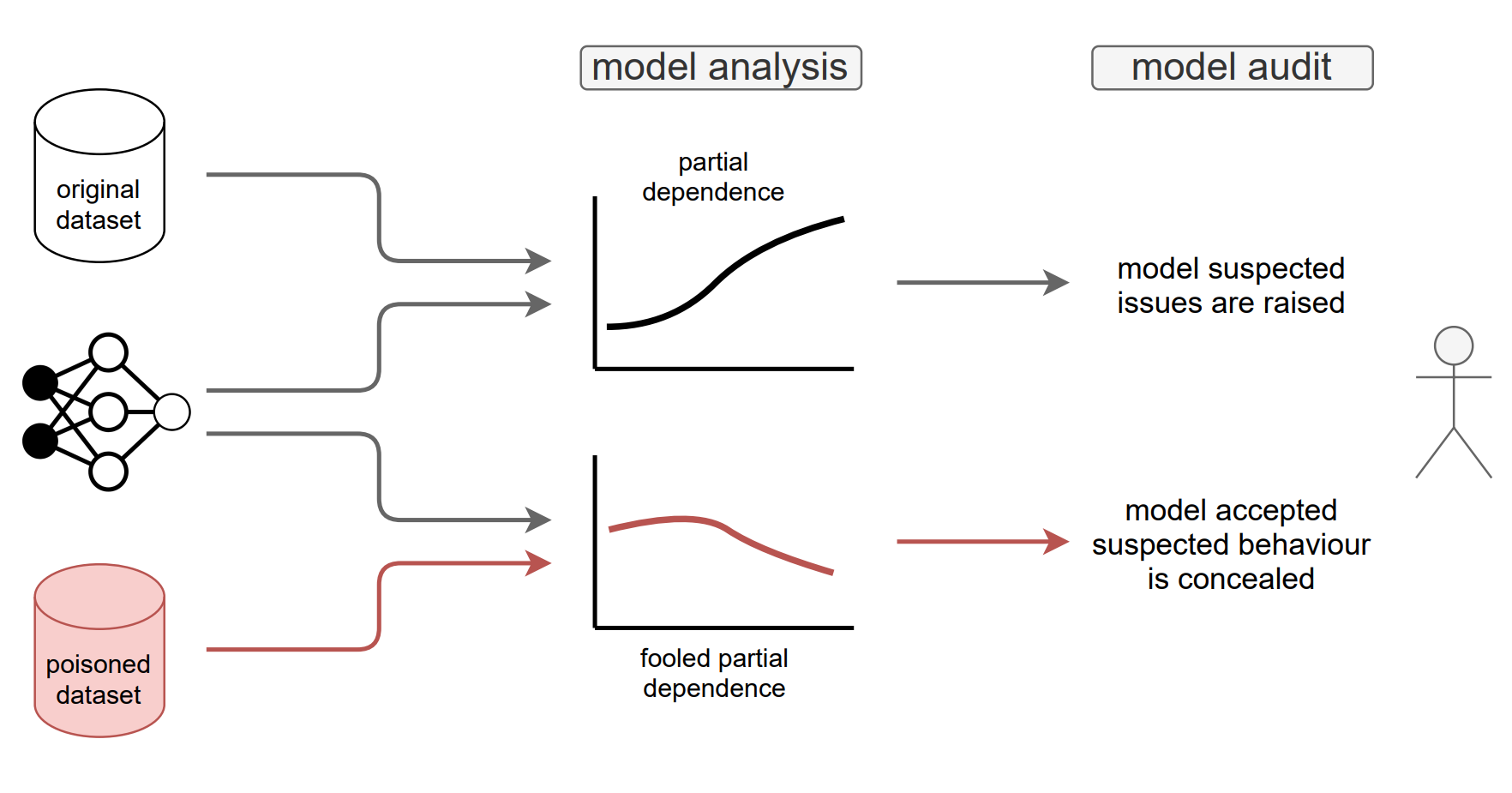
Hubert Baniecki, Wojciech Kretowicz, Przemyslaw Biecek
ECML PKDD (2022)
We showcase that PD can be manipulated in an adversarial manner, which is alarming, especially in financial or medical applications where auditability became a must-have trait supporting black-box machine learning. The fooling is performed via poisoning the data to bend and shift explanations in the desired direction using genetic and gradient algorithms.
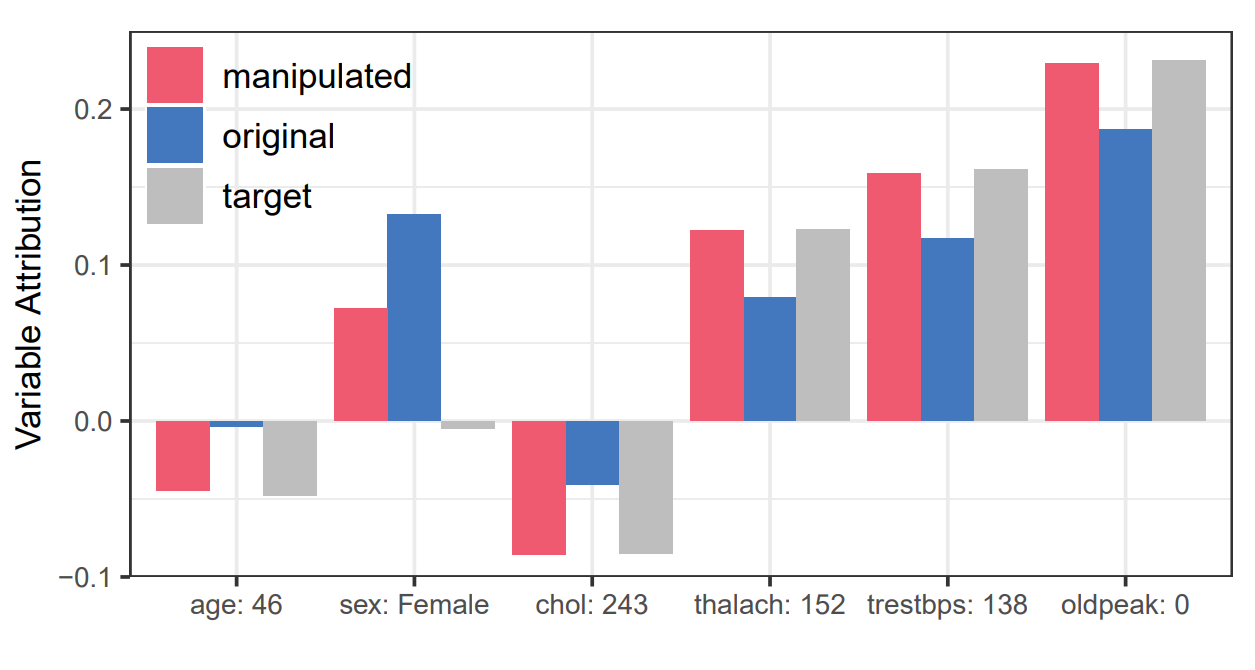
Hubert Baniecki, Przemyslaw Biecek
AAAI Conference on Artificial Intelligence (2022)
We introduce a model-agnostic algorithm for manipulating SHapley Additive exPlanations (SHAP) with perturbation of tabular data. It is evaluated on predictive tasks from healthcare and financial domains to illustrate how crucial is the context of data distribution in interpreting machine learning models. Our method supports checking the stability of the explanations used by various stakeholders apparent in the domain of responsible AI; moreover, the result highlights the explanations’ vulnerability that can be exploited by an adversary.
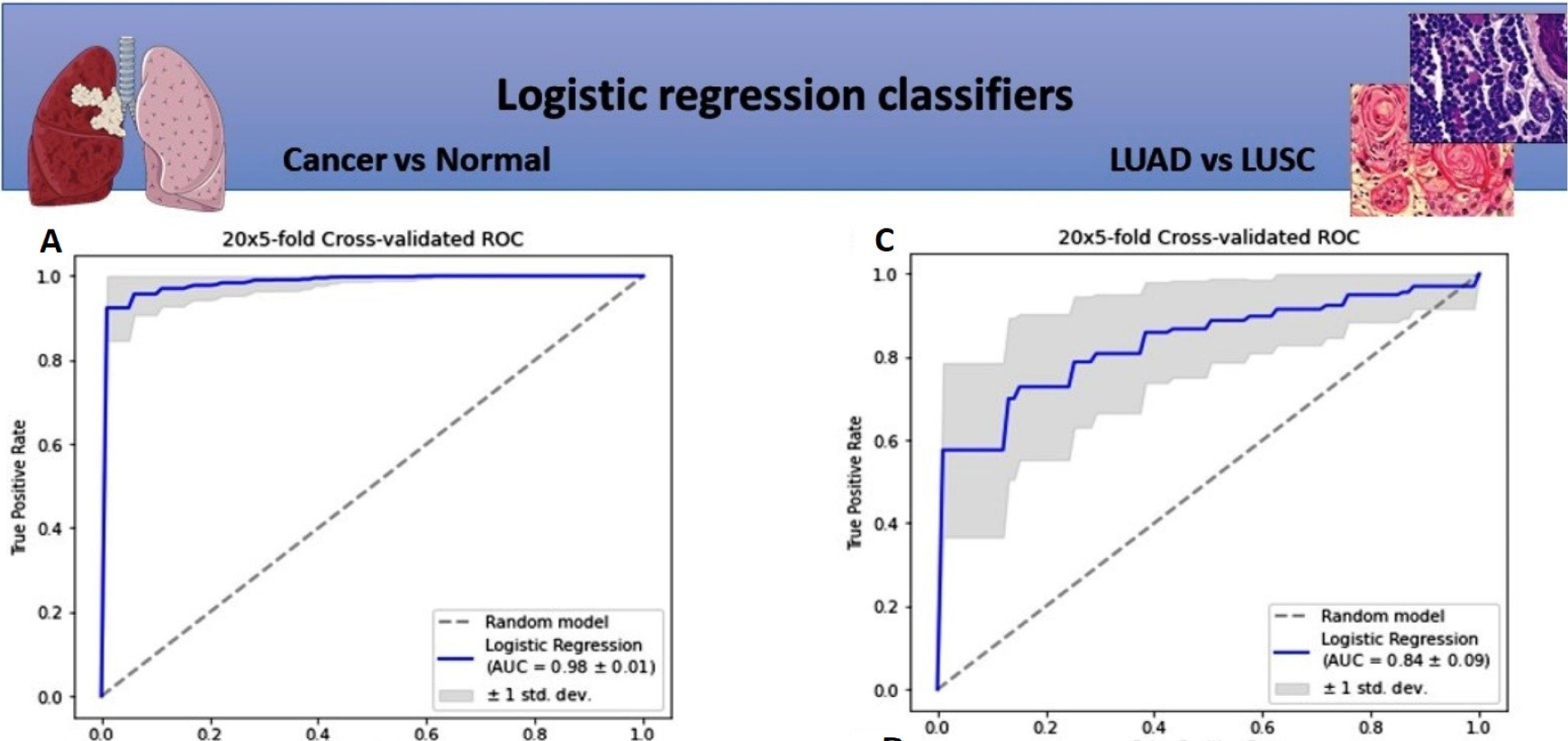
Anetta Sulewska, Jacek Niklinski, Radoslaw Charkiewicz, Piotr Karabowicz, Przemyslaw Biecek, Hubert Baniecki, Oksana Kowalczuk, Miroslaw Kozlowski, Patrycja Modzelewska, Piotr Majewski et al.
Cancers (2022)
The aim of the study was the appraisal of the diagnostic value of 14 differentially expressed long non-coding RNAs (lncRNAs) in the early stages of non-small-cell lung cancer (NSCLC). We established two classifiers. The first recognized cancerous from noncancerous tissues, the second successfully discriminated NSCLC subtypes (LUAD vs. LUSC). Our results indicate that the panel of 14 lncRNAs can be a promising tool to support a routine histopathological diagnosis of NSCLC.
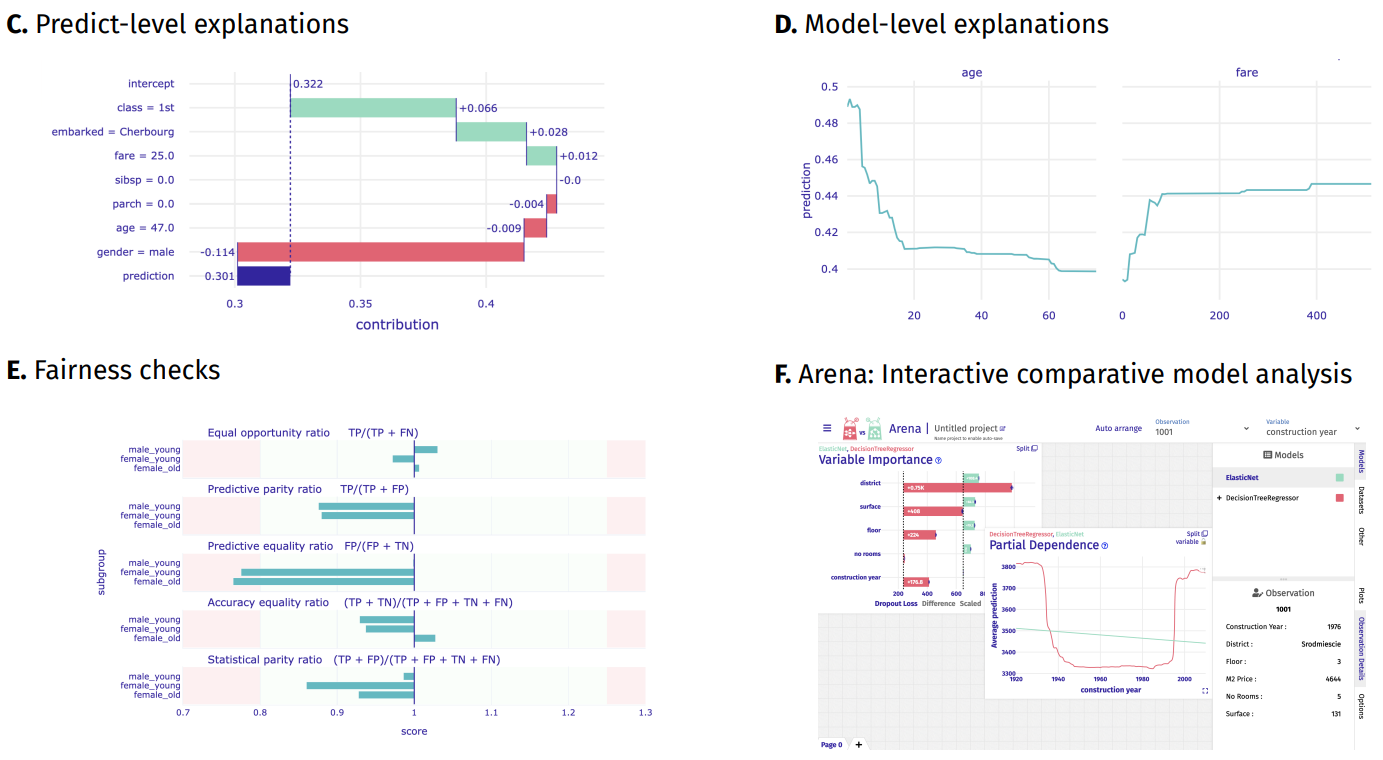
Hubert Baniecki, Wojciech Kretowicz, Piotr Piątyszek, Jakub Wiśniewski, Przemyslaw Biecek
Journal of Machine Learning Research (2021)
We introduce dalex, a Python package which implements a model-agnostic interface for interactive explainability and fairness. It adopts the design crafted through the development of various tools for explainable machine learning; thus, it aims at the unification of existing solutions. This library’s source code and documentation are available under open license at this URL.
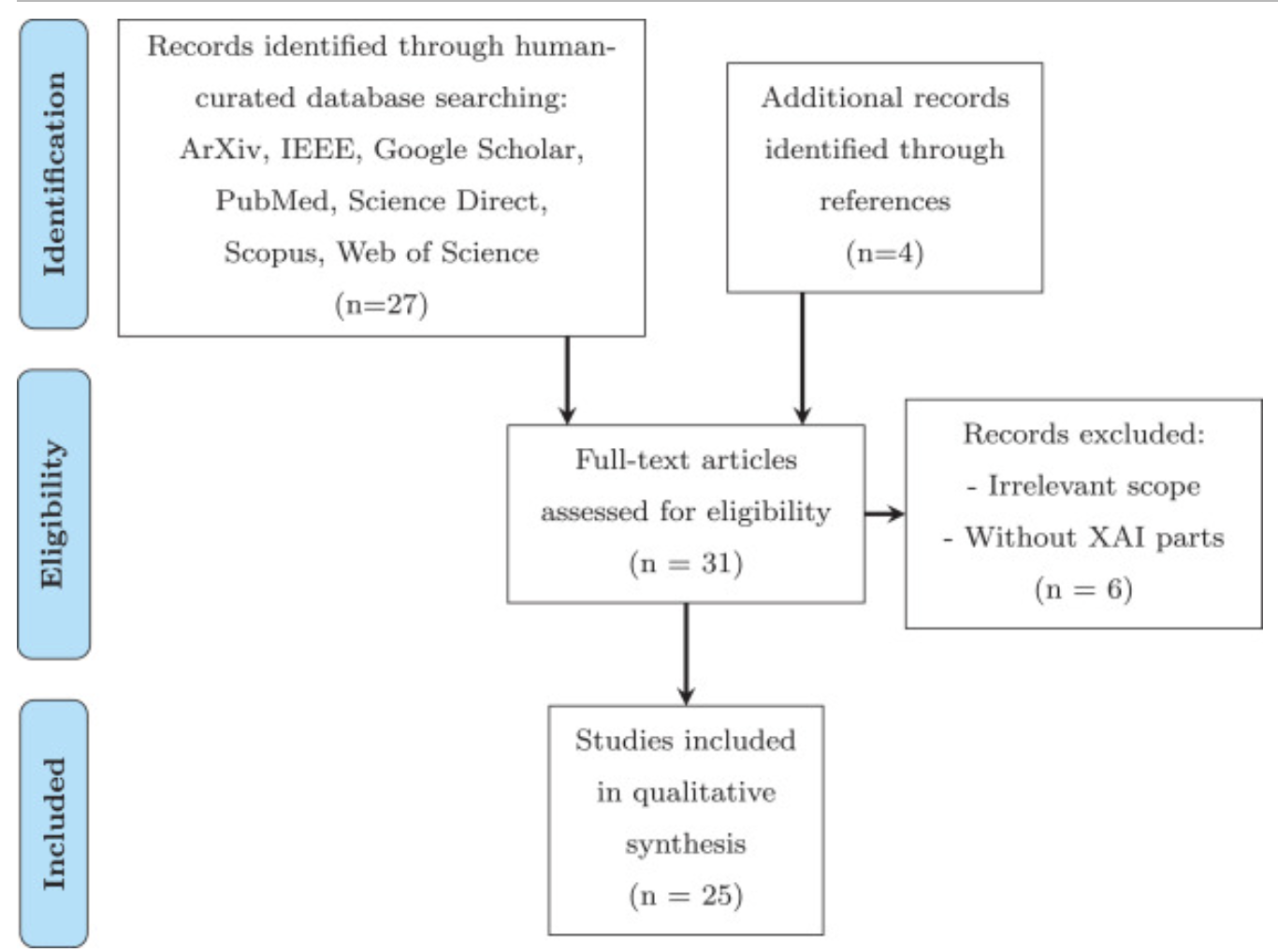
Weronika Hryniewska, Przemysław Bombiński, Patryk Szatkowski, Paulina Tomaszewska, Artur Przelaskowski, Przemysław Biecek
Pattern Recognition (2021)
Our analysis revealed numerous mistakes made at different stages of data acquisition, model development, and explanation construction. In this work, we overview the approaches proposed in the surveyed Machine Learning articles and indicate typical errors emerging from the lack of deep understanding of the radiography domain. The final result is a proposed checklist with the minimum conditions to be met by a reliable COVID-19 diagnostic model.
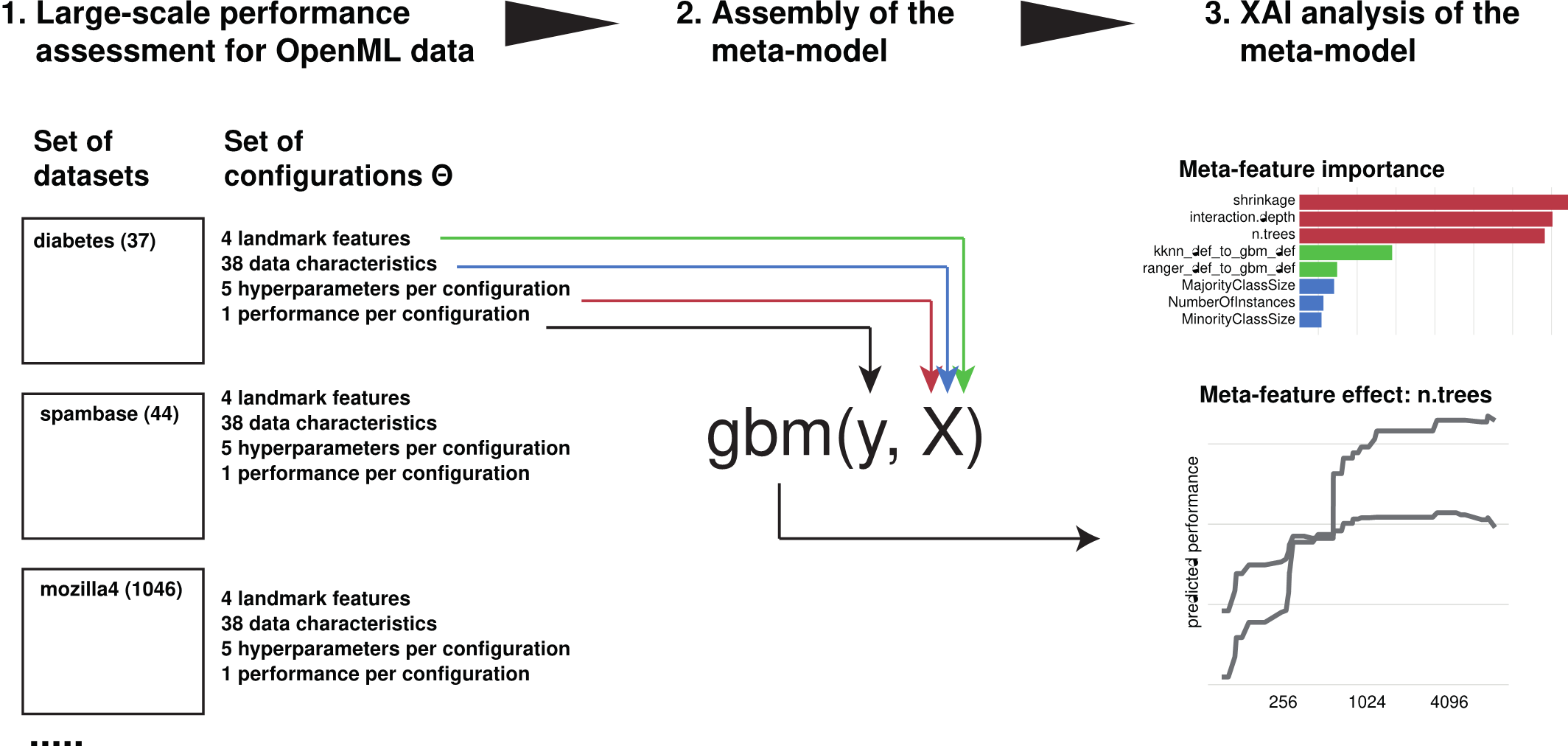
Katarzyna Woźnica, Przemyslaw Biecek
ECML PKDD Workshop on eXplainable Knowledge Discovery in Data Mining (2021)
To build a new generation of meta-models we need a deeper understanding of the importance and effect of meta-features on the model tunability. In this paper, we propose techniques developed for eXplainable Artificial Intelligence (XAI) to examine and extract knowledge from black-box surrogate models. To our knowledge, this is the first paper that shows how post-hoc explainability can be used to improve the meta-learning.
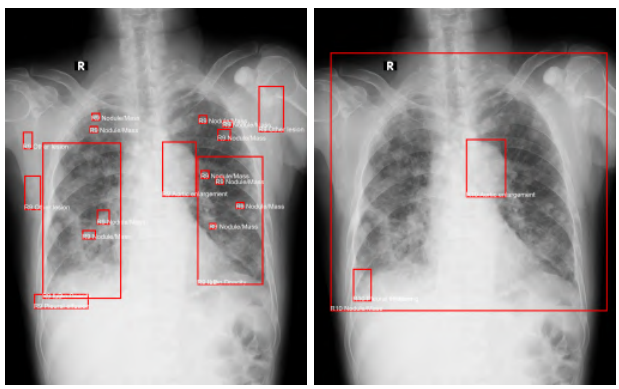
Weronika Hryniewska, Piotr Czarnecki, Jakub Wiśniewski, Przemysław Bombiński, Przemysław Biecek
CVPR Workshop on “Beyond Fairness: Towards a Just, Equitable, and Accountable Computer Vision” (2021)
In this paper, we analyze in detail a single use case - a Kaggle competition related to the detection of abnormalities in X-ray lung images. We demonstrate how a series of simple tests for data imbalance exposes faults in the data acquisition and annotation process. Complex models are able to learn such artifacts and it is difficult to remove this bias during or after the training.
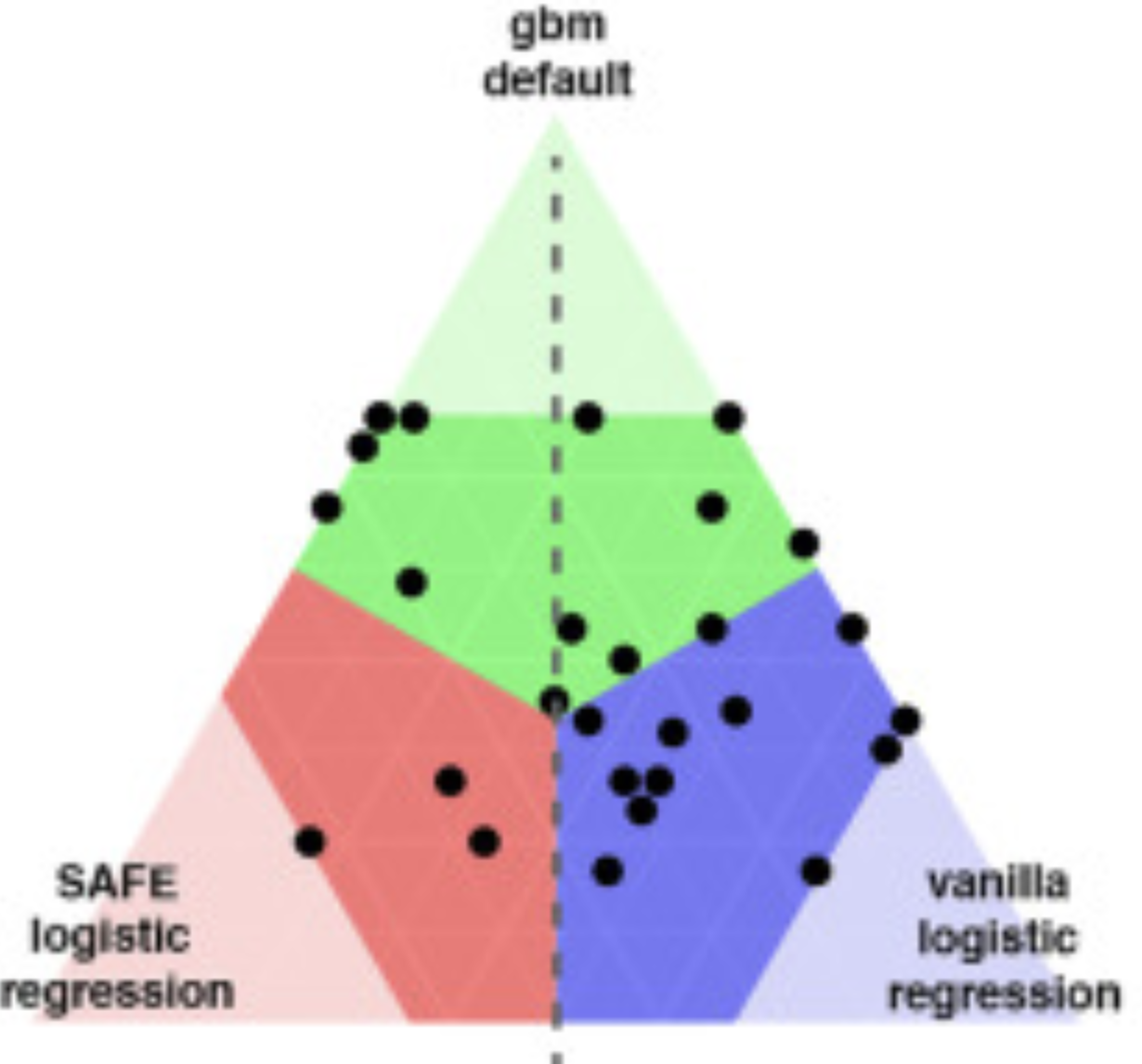
Simpler is better: Lifting interpretability-performance trade-off via automated feature engineering
Alicja Gosiewska, Anna Kozak, Przemysław Biecek
Decision Support Systems (2021)
We propose a framework that uses elastic black boxes as supervisor models to create simpler, less opaque, yet still accurate and interpretable glass box models. The new models were created using newly engineered features extracted with the help of a supervisor model. We supply the analysis using a large-scale benchmark on several tabular data sets from the OpenML database.
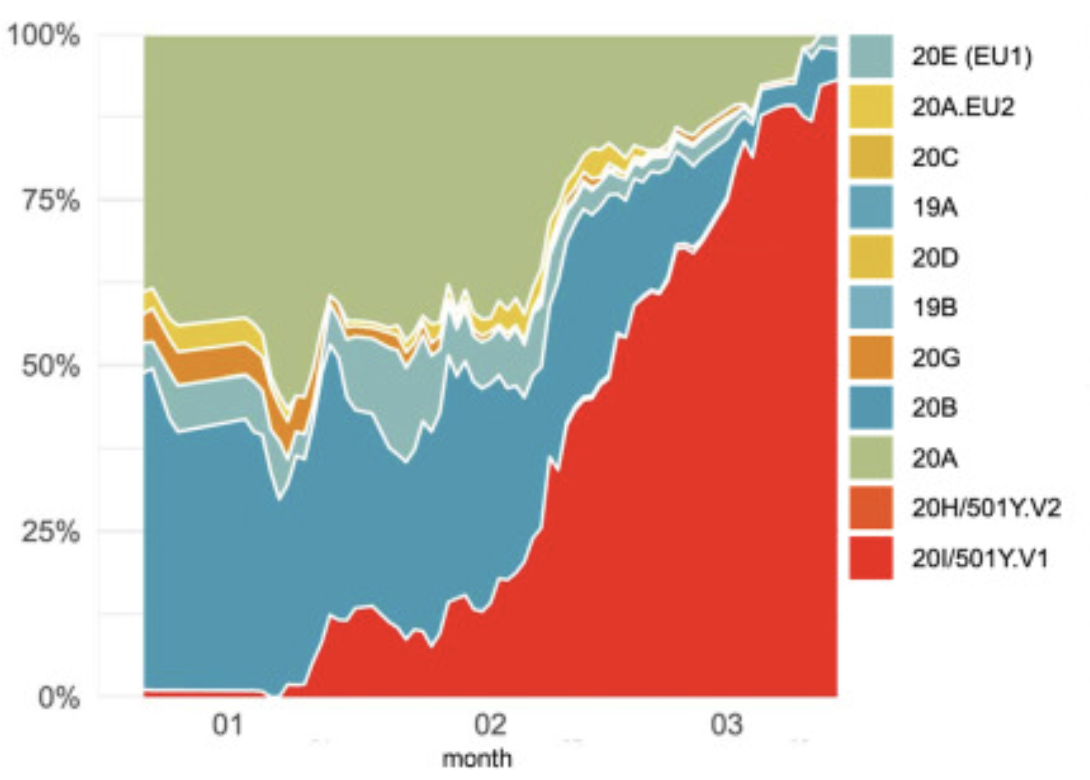
Radosław Charkiewicz, Jacek Nikliński, Przemysław Biecek, Joanna Kiśluk, Sławomir Pancewicz, Anna Moniuszko-Malinowska, Robert Flisiak, Adam Krętowski, Janusz Dzięcioł, Marcin Moniuszko, Rafał Gierczyński, Grzegorz Juszczyk, Joanna Reszeć
Advances in Medical Sciences (2021)
This study shows the first confirmed case of SARS-CoV-2 in Poland with the lineage B.1.351 (known as 501Y.V2 South African variant), as well as another 18 cases with epidemiologically relevant lineage B.1.1.7, known as British variant.
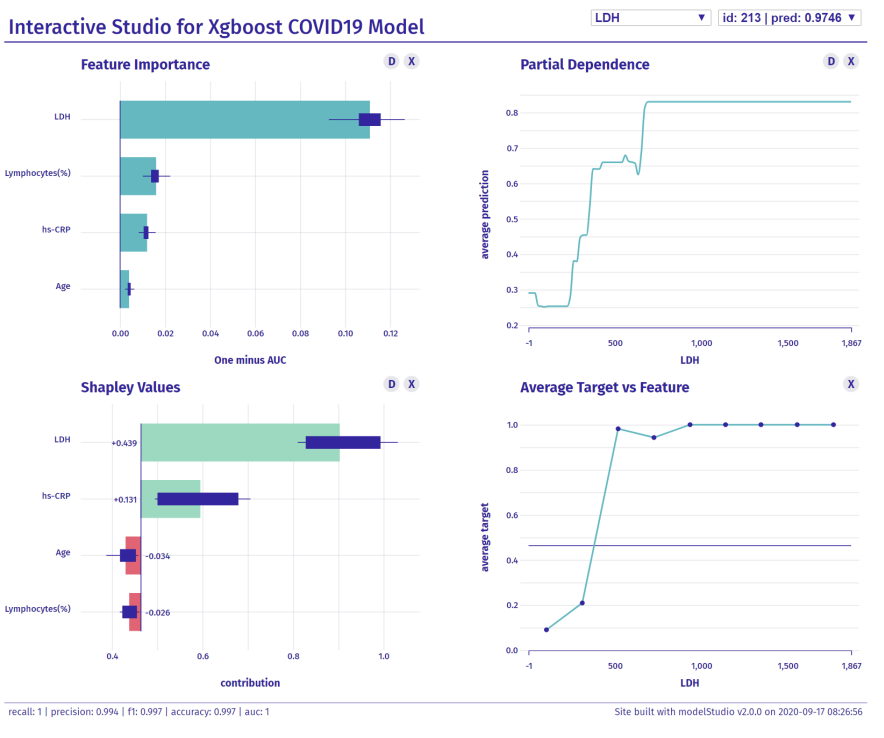
Hubert Baniecki, Przemyslaw Biecek
AAAI Conference on Artificial Intelligence (2021)
During the literature review of COVID-19 related prognosis and diagnosis, we found out that most of the predictive models are not faithful to the RAI principles, which can lead to biassed results and wrong reasoning. To solve this problem, we show how novel XAI techniques boost transparency, reproducibility and quality of models.
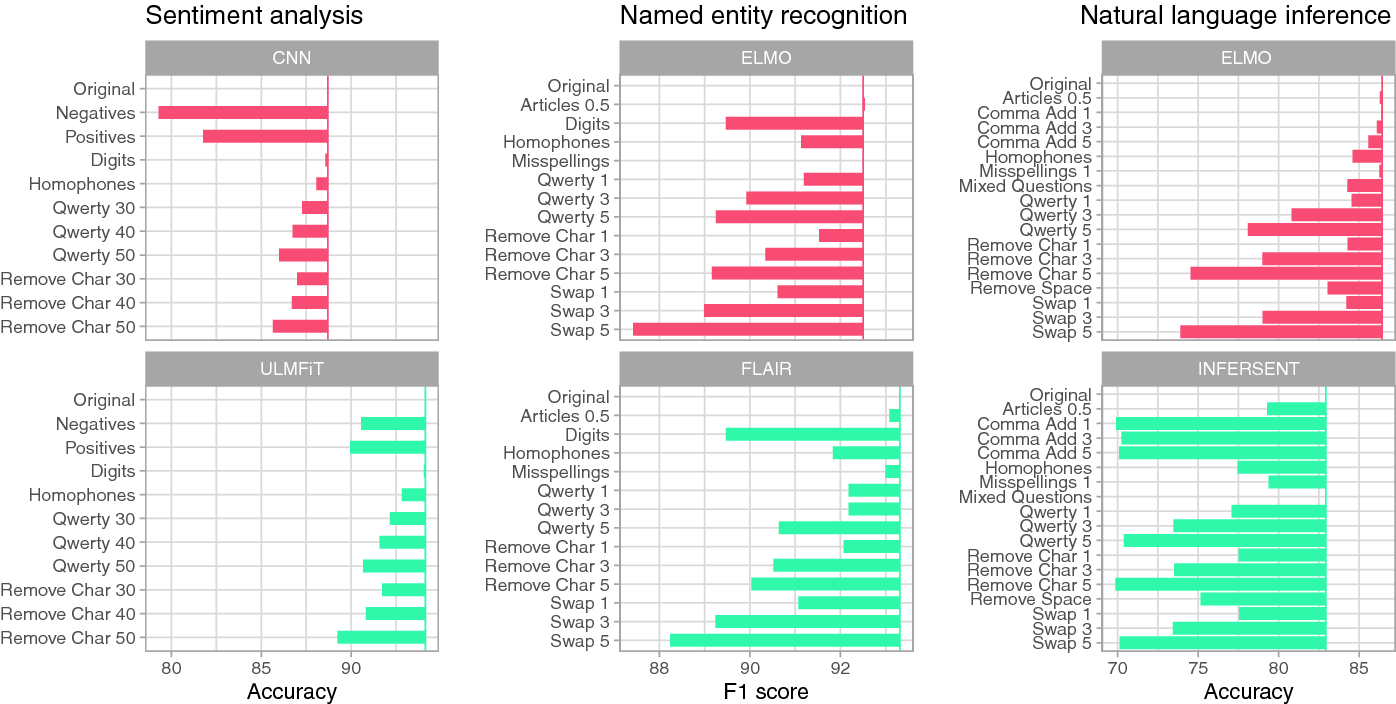
Barbara Rychalska, Dominika Basaj, Alicja Gosiewska, Przemyslaw Biecek
International Conference on Neural Information Processing (2019)
In this paper we introduce WildNLP - a framework for testing model stability in a natural setting where text corruptions such as keyboard errors or misspelling occur. We compare robustness of deep learning models from 4 popular NLP tasks: Q&A, NLI, NER and Sentiment Analysis by testing their performance on aspects introduced in the framework. In particular, we focus on a comparison between recent state-of-the-art text representations and non-contextualized word embeddings. In order to improve robustness, we perform adversarial training on selected aspects and check its transferability to the improvement of models with various corruption types. We find that the high performance of models does not ensure sufficient robustness, although modern embedding techniques help to improve it.
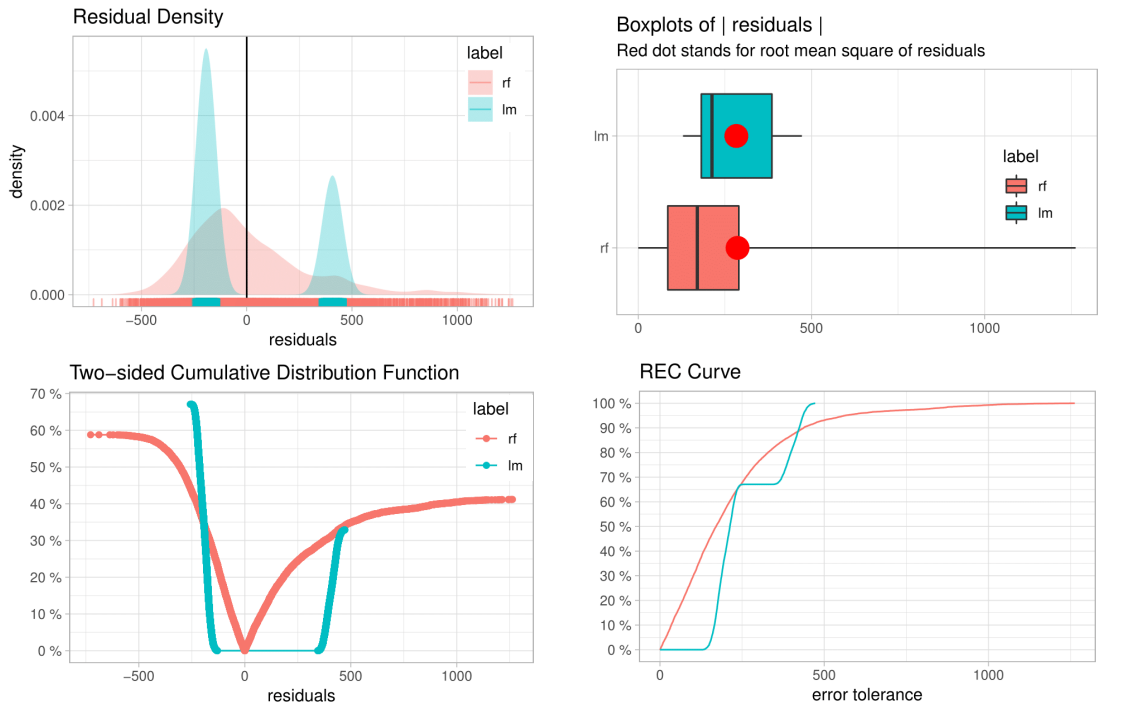
Alicja Gosiewska, Przemyslaw Biecek
The R Journal (2019)
This paper describes methodology and tools for model-agnostic auditing. It provides functinos for assessing and comparing the goodness of fit and performance of models. In addition, the package may be used for analysis of the similarity of residuals and for identification of outliers and influential observations. The examination is carried out by diagnostic scores and visual verification. The code presented in this paper are implemented in the auditor package. Its flexible and consistent grammar facilitates the validation models of a large class of models.
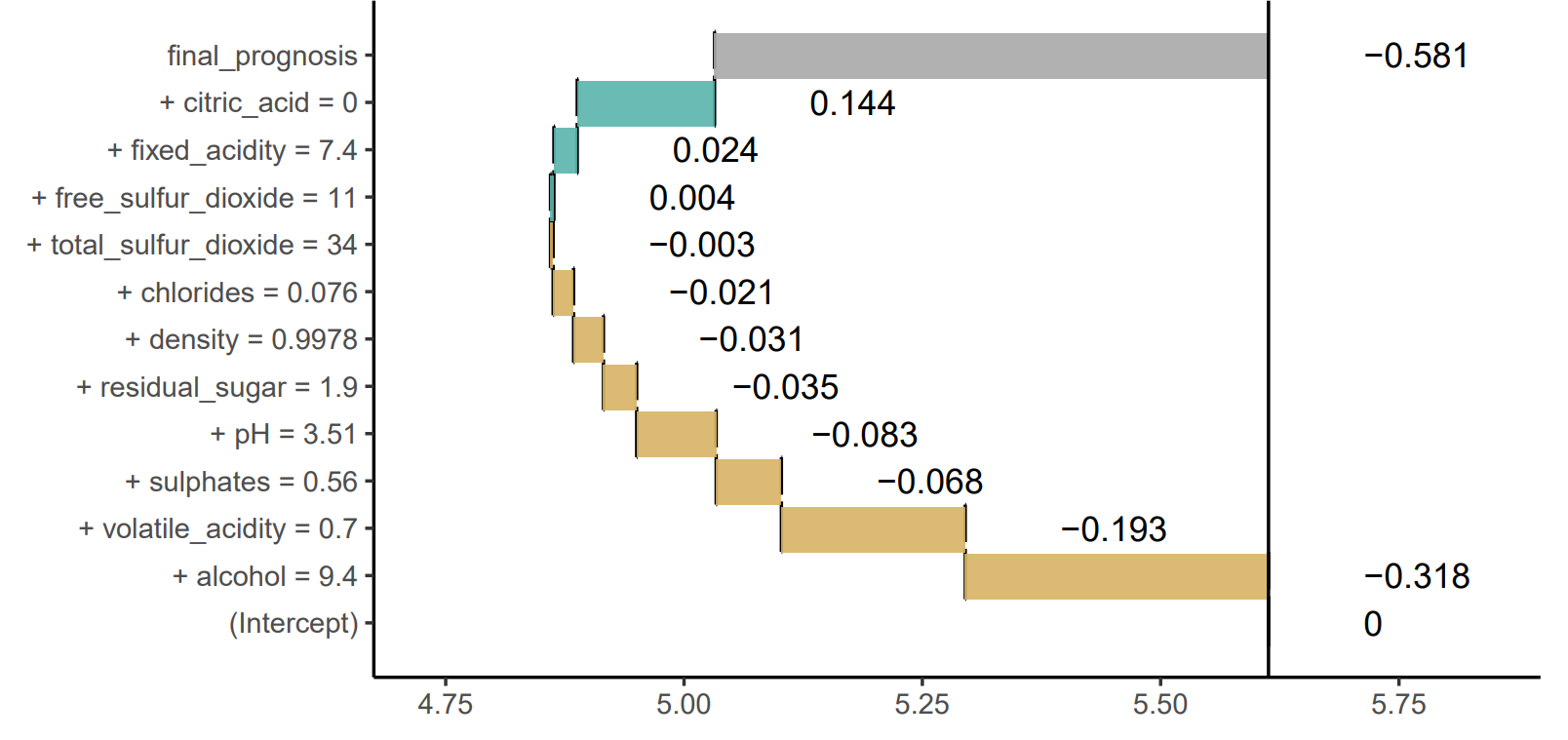
Mateusz Staniak, Przemyslaw Biecek
The R Journal (2018)
Complex models are commonly used in predictive modeling. In this paper we present R packages that can be used for explaining predictions from complex black box models and attributing parts of these predictions to input features. We introduce two new approaches and corresponding packages for such attribution, namely live and breakDown. We also compare their results with existing implementations of state-of-the-art solutions, namely, lime (Pedersen and Benesty, 2018) which implements Locally Interpretable Model-agnostic Explanations and iml (Molnar et al., 2018) which implements Shapley values.
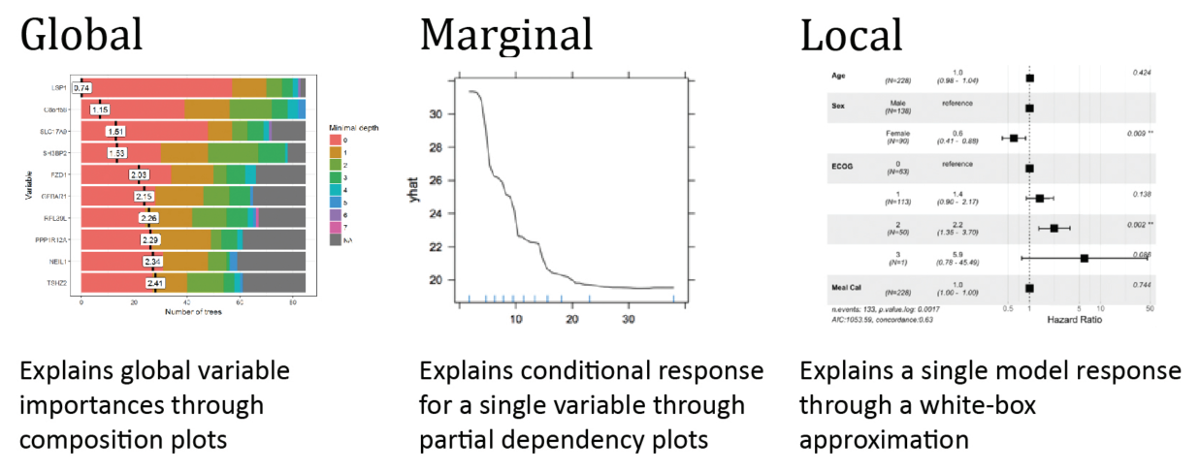
Przemyslaw Biecek
Journal of Machine Learning Research (2018)
This paper describes a consistent collection of explainers for predictive models, a.k.a. black boxes. Each explainer is a technique for exploration of a black box model. Presented approaches are model-agnostic, what means that they extract useful information from any predictive method irrespective of its internal structure. Each explainer is linked with a specific aspect of a model. Every explainer presented here works for a single model or for a collection of models. In the latter case, models can be compared against each other. Presented explainers are implemented in the DALEX package for R. They are based on a uniform standardized grammar of model exploration which may be easily extended.
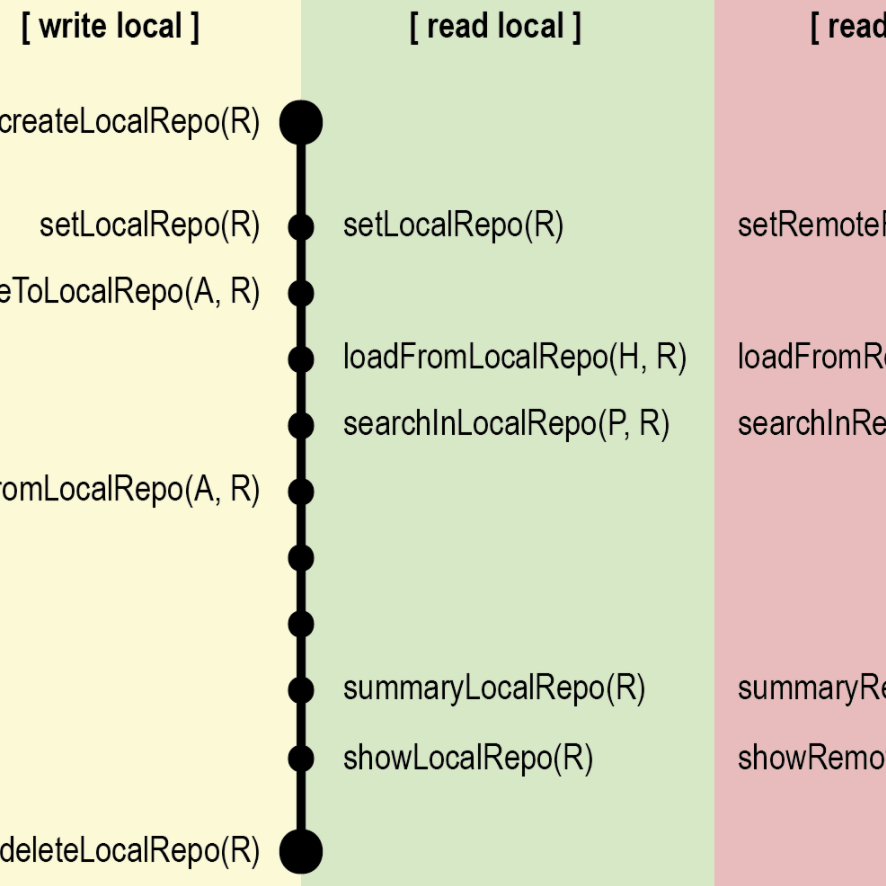
Przemyslaw Biecek, Marcin Kosiński
Journal of Statistical Software (2017)
Everything that exists in R is an object (Chambers 2016). This article examines what would be possible if we kept copies of all R objects that have ever been created. Not only objects but also their properties, meta-data, relations with other objects and information about context in which they were created. We introduce archivist, an R package designed to improve the management of results of data analysis.